Patents are essential to protect intellectual property in the rapidly changing technological landscape. The fundamental part of patent law is the drafting of a patent. This plays a crucial role in protecting these rights. This involves describing an invention in detail and precisely, and arguing why it should be protected.
As we enter the digital era, neural networks are becoming powerful tools that are reshaping many industries. The impact of neural networks has been felt in many industries, from healthcare to finance. Intellectual property law is not an exception.
This comprehensive exploration explores the fascinating world of neural networks and their role in the drafting of patents. We will explore the technology’s layers, its applications and the impact on intellectual property law.
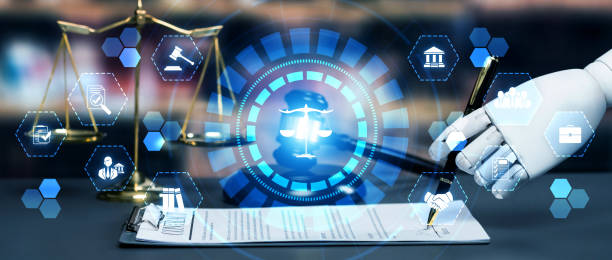
Understanding Patent Drafting
Let’s lay a solid foundation before we dive into the world neural networks by learning what patent drafting is all about.
What Is Patent Drafting?
Patent drafting is a process of translating the inventions of an inventor into a document that can be legally enforced. The patent application is a blueprint for the invention. This document provides a detailed description of the invention, its novelty and potential benefits.
Patent applications are composed of several important components.
1. Title: The title is the name of the invention, and it gives a concise description of what the patent covers.
2. Abstract: Brief summary of invention, highlighting key features and benefits.
3. Specification. This section is the heart of the application and provides a detailed description of the invention, including its structure, function, operation, etc.
4. Claim: Probably the most important part of the application is the claims, which define the scope and content of the patent. They define what is protected, and what other people are prohibited from making or using, or selling.
What is the role of patent attorneys and agents?
Patent drafting requires a special skill. Inventors seek out the help of patent agents or attorneys to navigate through this complicated terrain. These professionals have a thorough understanding of patent law and ensure that the application meets the strict requirements set by the patent office.
Challenges in Patent Drafting
Patent drafting is important for protecting intellectual properties, but it comes with its own challenges. A robust patent application requires a thorough understanding of the invention as well as the complexities of patent law. The application may be rejected or have its scope reduced if it contains ambiguities or mistakes.
The patenting process is time-consuming, expensive and can also be lengthy. It’s therefore important to improve the efficiency and streamline the process. Neural networks are a promising solution that could revolutionize patent drafting.
Let’s first trace the history of patent writing technology.
The Evolution of Technology in Patent Drafting
In the past, drafting a patent was a laborious and meticulous process. Patent attorneys would carefully examine technical details to ensure that the application was complete and error-free. The advent of technology has gradually changed this landscape.
Traditional Methods for Patent Drafting
In the past, patent preparation was heavily reliant on manual research. In the past, attorneys and agents were required to search through large libraries of prior art to find relevant references in order to prove the novelty of an invention. The process was time-consuming and prone to human error. It was often the ability of the attorney to locate and cite relevant prior art that determined the success of a new patent application.
The Emergence of Technology for Patent Drafting
Patent professionals have become more sophisticated as technology has advanced. The move from paper-based documents to digital databases was a major step forward. Online patent databases made it easier to access prior art and streamline the research phase. Computer software began to be used in the drafting of applications. It helped organize information and create patent documents. These advances paved the path for more efficient patent drafting.
Automation and Efficiency: The Need
Despite the fact that these technological advances brought about notable improvements, there is still a demand for more automation and efficiency. Patent applications were increasing, driven by the need to protect intellectual properties and innovation. Patent professionals were looking for a solution to speed up the drafting process, without compromising on quality.
This is where neural network technology comes into play. These AI-driven patent systems will revolutionize the patent writing process by harnessing artificial intelligence and machine learning.
Neural Networks: An Overview
Let’s make sure we understand what neural networks are, and how they work.
What are neural networks?
The neural network is a subset in artificial intelligence that was inspired by the structure and function of the brain. They are based on interconnected processing units known as neurons. They work together, just like our brain’s neurons, to process information, recognize pattern, and make predictions.
The Human Brain Mimicked
The remarkable learning and adaptation abilities of the brain are mirrored by neural networks. They are particularly good at tasks involving pattern recognition such as speech and image recognition, natural-language processing, and decisions.
Layers of neurons interconnected are the building blocks of neural network. These layers include:
- Input layer: Receives information in the form text, images or numeric values.
- Hidden layers: Intermediate layer between the input and output layers where complex calculations occur.
- Output layer: Produces final results or predictions.
In order to make sense of the data, neural networks adjust connections between neurons. This process is known as training. Training helps neural networks recognize patterns and predict based on data input.
Let’s now explore the various types of neural network relevant to patent writing.
Types and Applications of Neural Networks in Patent Drafting
There are many different types of neural networks, each one suited for a specific task. In the context for patent drafting, neural networks of various types play a pivotal role:
- Feedforward Networks: This is the simplest neural network, where information flows in one direction from input to output. These networks are often used for tasks such as image classification and regression analyses.
- Convolutional Neural Networks: CNNs were designed to handle tasks that involve grid-like data such as images or videos. They are excellent at image recognition, and they have been used in patent-related images analysis.
- Recurrent neural networks (RNNs). RNNs can be used for natural language tasks and are especially useful when dealing with sequential data. They are therefore invaluable for patent drafting.
- Generative adversarial networks (GANs). A GAN is made up of two neural network, a discriminator and a generator, that are engaged in a competition. They were used to generate patent-like illustrations and documents.
Let’s now explore the applications of neural networks in patent drafting.
Neural networks in patent search and prior art analysis
A thorough patent search and prior-art analysis is one of the most important aspects of patent preparation. Patent professionals need to identify patents and publications which are similar to the invention. This step is essential to establishing the novelty and non-obviousness of the invention.
The role of neural networks in patent search
Patent databases are often searched using keywords. Neural networks have revolutionized the process. They can:
- Semantic search: Neural network semantic search allows for identification of prior art and patents based on meaning and context, not just keywords.
- Image Based Search: When inventions include visual elements such as product designs or patent drawings, neural networks can match images and patent drawings to find relevant prior arts.
Enhancing Prior Art Analyses with Neural Networks
Analyzing the prior art requires assessing the similarity and relevance of existing patents with the invention in question. In this process, neural networks help by:
- Natural Language Processing: Neural network equipped with NLP capability can analyze textual content in patents to identify relevant sections and claims.
- Semantic Analyses: The neural networks excel at semantic analysis and help patent professionals to determine the similarity of the invention with existing patents, based on the technical content.
Neural networks in Natural Language Processing (NLP).
Natural Language Processing (NLP) is a branch of artificial intelligence that focuses on the interaction between computer and human language. NLP is crucial in the context of drafting patents, as it helps to understand and process textual information.
The Importance of NLP for Patent Drafting
Patents require the analysis of vast quantities of textual information. Patent documents, legal texts and technical literature are all included. NLP allows computers to interpret and create human language. This makes it an invaluable tool for patent drafting.
- Patent Search: Using NLP algorithms, search queries can be processed in natural language to retrieve patent documents with greater accuracy than keyword searches.
- Prior Art Analyses: NLP is used to compare and understand the content of patent documents in order to identify similarities, differences, or potential infringements.
- Automated Summary: NLP driven summarization tools are able to condense long patent documents into concise summaries. This makes it easier for professionals to review and analyze.
- Language translation: Natural language processing (NLP) can help translate patent documents from one foreign language to another. This will facilitate global filing and analysis of patents.
How neural networks enhance NLP for patent-related tasks
In the context of patent writing, neural networks are a significant improvement in NLP, thanks to their ability for processing and understanding complex patterns. Neural Networks excel at capturing subtle nuances and context in patent documents. This allows for a more accurate determination to the relevance of prior arts and identification of key concepts.
In contrast to traditional NLP models, which often struggle with word ambiguity and are unable to understand the context of words, neural networks have demonstrated remarkable ability in understanding this context. This results in a more accurate and context-aware understanding of natural language.
Neuronal networks can classify and identify entities like inventors’ names and company names within patent documents. This helps extract valuable information. The ability of neural networks to understand complex sentences and their dependencies is used in parsing patent claims.
Use of neural networks and NLP in patent drafting
Patent drafting has benefited from the fusion of NLP with neural networks.
AI powered tools can analyze and extract key information from patent documents and evaluate the strength and validity of the patent. NLP & neural networks help manage large patent portfolios through categorizing patents, tracking changes to patent laws, and monitoring of competitors.
Global Patent filing often requires translations in multiple languages. Translation services are provided by neural networks, which offer accurate and efficient translation. Some AI-based systems can automatically generate patent applications, or draft specific sections in patent documents, based on input data. The incorporation of neural networks in NLP-driven processes for patent drafting enhances accuracy and efficiency. It also allows valuable insights to be uncovered from vast amounts of textual data.
Neural Networks in Patent Classification and Categorization
Patent classification is the process of organizing patents into specific categories based on their subject matter, technology, or industry. These classifications serve multiple purposes, including facilitating patent search, providing insights into technological trends, and aiding in the examination of patent applications. Traditionally, patent classification has been performed manually by patent examiners, a labor-intensive and time-consuming task.
However, the advent of neural networks and machine learning has introduced automation and efficiency into patent classification processes. Several organizations and research institutions have developed neural network-based patent categorization systems:
Google Patents: Google’s patent search and analysis tool utilizes machine learning algorithms, including neural networks, to categorize patents and make them more accessible to users. It also provides visualization tools to explore patent landscapes.
WIPO’s AI-Powered Patent Classification: The World Intellectual Property Organization (WIPO) employs artificial intelligence, including neural networks, to classify patents into its International Patent Classification (IPC) system more efficiently.
IBM’s Watson for Patents: IBM’s Watson, powered by deep learning, offers patent classification services, assisting patent professionals in quickly identifying relevant patents.
These examples demonstrate the real-world impact of neural network-driven patent categorization systems, simplifying the patent search and analysis process.
Neural networks, with their ability to automate and enhance patent classification and categorization, are instrumental in managing the vast and intricate world of patent data. They not only increase efficiency but also contribute to more comprehensive and accurate patent analysis.
Neural networks in Patent Drafting Assistance
Patent drafting, as we have seen, is a meticulous and complex process. It requires an in-depth understanding of technical details and legal nuances. Even for experienced attorneys, drafting patent applications can be time-consuming. The use of neural networks in patent drafting has become a powerful tool. They offer a variety of capabilities that can streamline and improve the drafting process.
Automating Patent Drafting Tasks
Neural network, in particular language models such as GPT-3 can generate patent drafts, or specific sections of a patent application based on the information provided. This can speed up the writing process.
Patent claims are critical for defining scope of protection and can be automated by neural networks. These models can generate or suggest claims based on an invention’s description. Certain neural networks are able to generate technical illustrations and diagrams based on descriptions in text, making it easy to visualize complex inventions.
Improving Patent Quality
Neural Networks can analyze and improve the language used in Patent Applications to ensure clarity and accuracy. This is especially useful when dealing with technical jargon. Neural Networks can be used to check for compliance with patent guidelines and requirements.
Enhancing search and prior art analysis
Neuronal networks can help patent professionals identify relevant prior art early in the writing process. Integrating neural network-powered semantic search capabilities in patent drafting tools enables more comprehensive searches of relevant prior art.
Limitations & Ethical Considerations
It is important to understand the limitations of neural networks and their ethical implications when drafting patents. Neural network accuracy is not perfect and it can make mistakes, especially when the input data are incomplete or biased.
Patent professional must use AI-assisted writing tools ethically. They should ensure that the AI-generated content accurately represents an invention and is compliant with patent laws. AI should enhance human expertise and not replace it. Patent professionals must use their critical judgement and legal expertise when reviewing and finalizing drafts of patents. To protect sensitive intellectual property, the use of neural networks as a patent drafting tool should comply with data privacy and security regulations.
The integration of neural network technology into patent drafting tools is a major step forward in intellectual property law. These tools improve efficiency, accuracy and collaboration, while allowing professionals to concentrate on the strategic aspects of their work.