Many financial firms are already using AI to accelerate insights, respond faster, and predict more accurately. However, there are also concerns that AI may amplify existing risks to financial stability.
As highly regulated entities, financial institutions and their suppliers must be aware of legal requirements that apply to the development, use and provision of AI. This includes ensuring the appropriate management of liability. Here are a few key ways that AI and machine-learning are used in financial regulations:
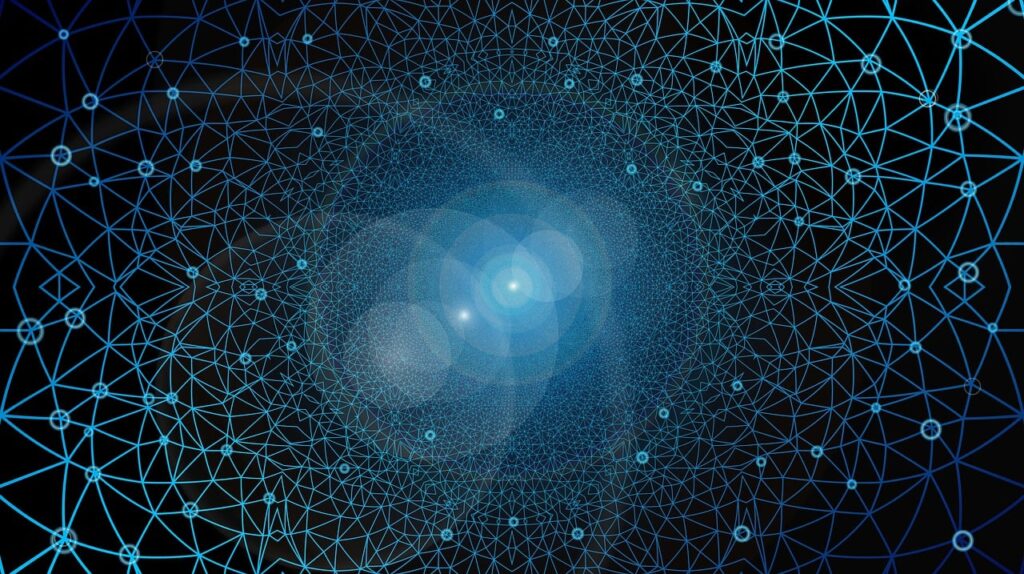
Risk assessment
AI algorithms and machine learning can analyze large amounts of financial data to predict and assess risks in real time. It is possible to identify market anomalies and fraud as well as assess the creditworthiness and financial standing of individuals and institutions. These technologies help regulators to proactively address systemic risk and prevent financial crisis.
AI and machine learning algorithms have revolutionized the field of risk assessment in finance by enabling more accurate, timely, and data-driven risk evaluations. Here’s a deeper discussion of how these technologies are transforming risk assessment in the financial industry:
1. Data Volume and Speed
Financial markets generate enormous amounts of data every second. AI and machine learning excel at processing and analyzing this data at speeds far beyond human capability. This enables real-time risk assessment, which is crucial in a fast-paced and volatile market environment.
2. Predictive Modeling
Machine learning algorithms can create predictive models based on historical data to forecast various types of risks. For example:
- Market Risk: Predicting market movements, volatility, and asset price fluctuations.
- Credit Risk: Assessing the creditworthiness of borrowers by analyzing their financial history, credit scores, and economic conditions.
- Operational Risk: Identifying vulnerabilities in internal processes, fraud detection, and errors that may lead to financial losses.
- Liquidity Risk: Evaluating the ability to meet short-term financial obligations by analyzing cash flow data.
3. Pattern Recognition
Machine learning algorithms excel at recognizing complex patterns and anomalies in data. They can detect irregular market behavior, fraudulent transactions, or abnormal trading activities that may indicate elevated risk.
AI-powered tools can optimize investment portfolios by balancing risk and return. They consider various risk factors, including sectoral exposure, asset correlation, and historical performance, to create diversified and risk-adjusted portfolios.
Machine learning models can simulate various economic scenarios and assess how different risk factors might affect a financial institution’s or portfolio’s performance under stress. This aids in identifying vulnerabilities and making informed decisions to mitigate risks.
4. Regulatory Compliance
AI can assist in ensuring regulatory compliance by monitoring and flagging potential violations. This is particularly useful in areas such as anti-money laundering (AML) and know your customer (KYC) checks, where large volumes of data need to be analyzed for suspicious activities.
Machine learning models can improve credit scoring by considering a wider range of data sources and variables. This allows for more accurate assessments of borrowers’ creditworthiness, reducing the risk of defaults.
5. Operational Efficiency
Automation through AI streamlines the risk assessment process. This reduces the need for manual data entry and analysis, minimizing human errors and saving time.
Challenges in AI-based risk assessment include the need for high-quality and unbiased data, model interpretability, and addressing ethical concerns. Additionally, the dynamic nature of financial markets requires continuous model adaptation and monitoring.
AI and machine learning are transforming risk assessment in finance by enabling more precise, real-time, and data-driven analyses. These technologies are helping financial institutions and regulators stay ahead of evolving risks and make more informed decisions to protect investments, ensure regulatory compliance, and enhance overall financial stability.
Surveillance
Regulating agencies use AI-powered systems for surveillance to monitor trading activity and detect market manipulators, insider traders, and other illegal actions. These systems are able to process large amounts of data in order to identify patterns and behaviors that warrant further investigation.
Financial Institutions are using AI to ensure that they comply with regulations, such as Anti-Money Laundering and Know Your Customers (KYC). Machine learning models are able to detect suspicious transactions and customers with greater accuracy than traditional rule-based methods.
Predictive Analysis
AI is a powerful tool that can predict market trends, risks and future events. Regulators can utilize predictive analytics to anticipate problems and take proactive actions to maintain the stability of financial markets.
Automation using AI can streamline the generation and submission of regulatory reports. This reduces errors and ensures financial institutions meet reporting requirements.
Fraud detection
The fight against fraud is becoming more intense in our digitally connected world. The fraudulent activities can take many forms. From credit card fraud to identity theft, insurance scams, and online phishing schemes. The sophistication of fraudsters is increasing, and so are the tools that can be used to fight them. Artificial Intelligence has become a powerful tool in the fight against fraudulent activity, changing the way that organizations prevent and detect fraud.
Artificial Intelligence has been instrumental in fraud detection and prevention. Its ability to analyze vast amounts of data, and identify patterns, that are often difficult for human analysts to detect, is a key factor. Here are a few key ways AI can make a difference.
a. Advanced Pattern Recognition
AI algorithms are capable of analyzing large datasets to detect subtle patterns that indicate fraudulent behavior. This includes identifying anomalies within transaction data such as irregular logins, unusual purchase patterns, or deviations in user behavior. As they gain experience, machine learning models adapt and become more accurate.
AI-powered systems are able to continuously monitor transactions in real-time and can detect suspicious behaviors quickly. Real-time analysis allows companies to take immediate actions to prevent fraud before it occurs.
b. Natural Language Processing
NLP algorithms analyze text data such as emails and chat messages. They are also used to analyze social media posts. These algorithms can detect fraudulent communications and phishing attacks by identifying suspicious contexts and language.
AI can analyze biometrics such as mouse movements, keystroke patterns, and voice patterns to verify identities. This ensures that the person behind a digital account or transaction really is who they say they are, and reduces identity theft.
c. Fraud Score Calculation
AI-driven fraud detection system assigns risk scores to transaction or user profiles. This allows organizations to prioritize response efforts. High-risk transactions are scrutinized more closely, while low-risk transactions can continue uninterrupted.
AI systems are able to continuously adapt and learn new data in order to stay ahead of fraud tactics. AI models can adapt quickly to new fraudster techniques.
d. Ethical and Challenges Considerations
AI can be a powerful tool to fight fraud. However, it does not come without challenges and ethical concerns. These include:
- Data Privacy: AI relies upon vast quantities of data, which often includes sensitive personal information. It is vital to safeguard this data in order to protect privacy and adhere with data protection laws.
- Fairness and Bias: AI models may unintentionally perpetuate biases that are present in the training data. This could lead to an unfair treatment of individuals or groups. AI fraud detection requires careful monitoring and mitigation to avoid bias.
- Adversarial attacks: Fraudsters have become more sophisticated as they try to avoid AI detection. Adversarial attacks may be used, in which fraudsters manipulate input data to trick AI systems. To counter these threats, it is necessary to test and improve continuously.
Algorithmic Trade Oversight
AI is a key component in improving oversight and regulatory compliance for algorithmic trading. Algorithmic Trading, fueled by complex algorithms, high-frequency strategies and trading techniques, has become an important component of the financial markets. It also poses serious risks, such as market manipulation and systemic instabilities. AI can assist in addressing these challenges through a variety of ways.
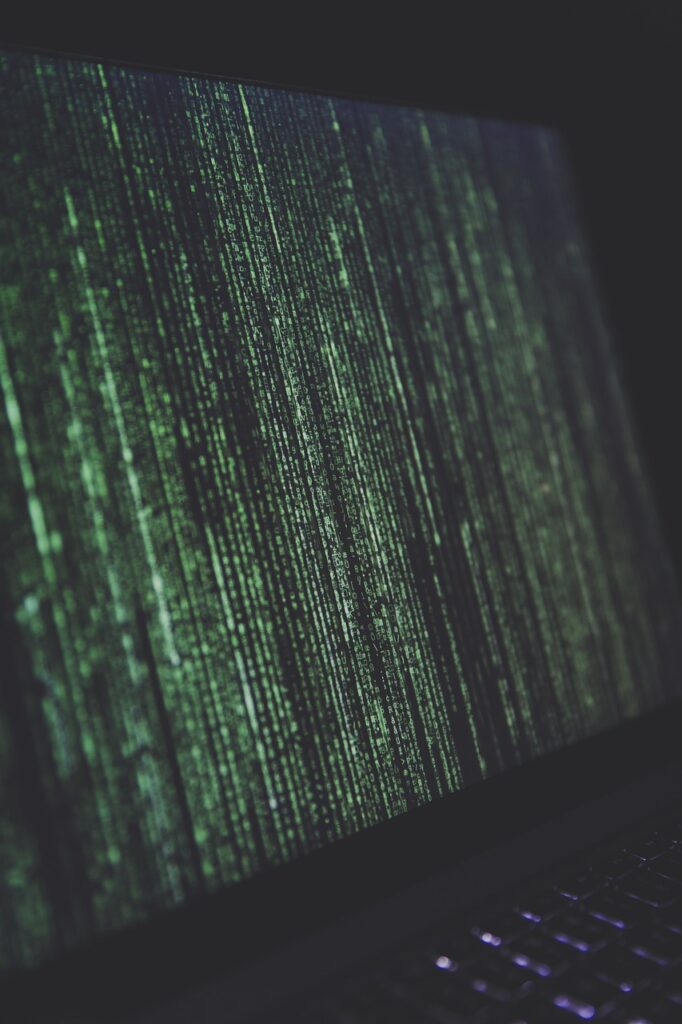
First, AI algorithms are able to continuously monitor trading activity in real-time. They can analyze vast amounts of data and detect patterns or irregularities that indicate market abuse. AI can help regulatory bodies enforce trading rules by identifying suspicious behavior.
AI can also improve the accuracy and efficiency of post-trade monitoring. It can analyze trade data, order flows, transaction records and other information automatically, ensuring trades are carried out within prescribed parameters. It reduces the need for manual review processes that are time-consuming and prone to errors.
A predictive analytics powered by AI can also forecast market anomalies and flash crashes based on historical data as well as current market conditions. This proactive approach allows regulators and participants in the market to take preventive measures to mitigate risks and avoid disruptions.
AI-powered Natural Language Processing (NLP), can also assist in monitoring social media sentiment and news that could impact trading decisions. It can identify relevant articles and social media posts quickly, gauge their sentiment and assess their potential impact on market sentiment. This helps traders and regulators make better decisions and stay informed.
AI is a valuable tool for algorithmic trading supervision. Not only does it improve real-time surveillance and post-trade monitoring, but it also allows for predictive analysis and sentiment tracking. In the age of algorithmic trading, market participants and regulatory bodies can protect the integrity and stability on financial markets by leveraging AI technology. It is important to make sure that AI systems are transparent, well-designed and updated regularly to keep up with market dynamics and new risks.
Consumer Protection
AI can be used for the analysis of consumer complaints to identify potential issues with financial products and services. This allows regulators to take the appropriate steps to protect consumers.
Machine-learning models can simulate different economic scenarios to assess how financial institutions will perform under stress. This allows regulators to assess the resilience of financial institutions and make informed decisions.
In order to implement AI and machine learning in financial regulation, there are several challenges. These include ensuring that AI models are transparent, fair, and interpreted correctly, as well as addressing privacy issues and keeping up with rapidly evolving financial technology. There is also a demand for highly skilled personnel who can deploy and manage AI systems within regulatory environments.
Overall, AI, machine learning, and other technologies have the potential to improve the effectiveness and efficiency of financial regulation. This will allow it to better respond to the complexity of the modern financial markets and the challenges that they pose.