Introduction
As artificial intelligence (AI) continues to advance and play an increasingly significant role in various domains, including healthcare, finance, and legal practice, ensuring ethical responsible governance and safe scaling of AI becomes imperative. This section explores the importance of ethical considerations, responsible governance frameworks, and the need for cautious scaling of AI technologies to mitigate risks and ensure the benefits are realized without compromising human values and societal well-being.
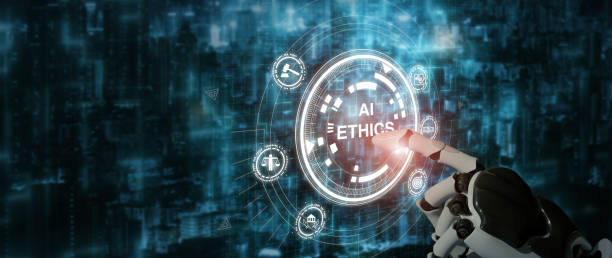
Ethical Considerations in AI Development and Deployment
Fairness and Bias
Fairness is a fundamental ethical consideration in AI development and deployment. AI algorithms learn from historical data, and if that data is biased, the algorithms can perpetuate or amplify existing biases. It is essential to identify and mitigate biases to ensure fair treatment and equal opportunities for all individuals. Key aspects of fairness and bias in AI include:
The Impact of Bias on AI Systems
Biases in AI systems can lead to discriminatory outcomes, reinforcing societal inequalities or exacerbating existing biases. It is crucial to recognize the potential for bias in data sources, algorithmic decision-making, and the deployment of AI systems.
Mitigating Bias in AI Algorithms
AI developers must take steps to identify and mitigate biases in AI algorithms. This can involve carefully curating training data to ensure representativeness and avoiding the reinforcement of discriminatory patterns. Regular audits and monitoring should be conducted to identify and rectify biases that may emerge during system deployment.
Ensuring Fairness in Decision-Making
AI algorithms should be designed to make fair decisions that do not discriminate based on protected characteristics such as race, gender, or religion. This requires developing fairness metrics, considering trade-offs between fairness and other performance measures, and evaluating the impact of AI systems on different demographic groups.
Transparency and Explainability
Transparency and explainability are crucial for ensuring accountability, trust, and ethical decision-making in AI systems. AI algorithms can be complex, making it challenging to understand their decision-making processes. However, the lack of transparency can lead to concerns about unfair or biased outcomes. Key considerations for transparency and explainability include:
- The importance of transparency in AI systems: Transparency allows users, regulators, and affected individuals to understand how AI systems work and make informed judgments about their use. Transparent AI systems engender trust, foster accountability, and enable users to assess the reliability and potential biases of the technology.
- Explainable AI and Interpretability: Efforts should be made to develop AI systems that are explainable and interpretable. This involves designing algorithms and models that can provide meaningful explanations for their decisions, allowing users to understand the reasoning behind AI-driven outcomes.
- Building Trust through Explainability: Explainability fosters trust in AI systems, particularly in high-stakes applications such as healthcare or legal decision-making. By providing clear explanations for the outputs of AI systems, users can better assess their reliability and make more informed decisions based on AI-generated insights.
Privacy and Data Protection
AI systems often rely on large amounts of data, including personal information, to train and operate effectively. Protecting privacy rights and ensuring data security are critical ethical considerations. Key aspects of privacy and data protection in AI development and deployment include:
- Addressing Privacy Concerns in AI Technologies: AI developers should prioritize privacy considerations throughout the development lifecycle. This includes ensuring compliance with applicable data protection regulations, implementing robust security measures to protect personal data, and obtaining informed consent for data usage.
- Safeguarding Sensitive Data: AI systems must handle sensitive data responsibly. Access to personal and sensitive information should be limited to authorized personnel, and data should be securely stored, encrypted, and anonymized whenever possible to protect individuals’ privacy rights.
- Complying with Data Protection Regulations: AI developers and deployers should adhere to relevant data protection regulations such as the General Data Protection Regulation (GDPR) or local privacy laws. This includes providing individuals with transparency and control over their data, implementing mechanisms for data breach notification, and respecting the rights of data subjects.
Accountability and Responsibility
Accountability and responsibility are vital considerations in AI development and deployment to ensure that the technology is used ethically and with proper oversight. Key aspects of accountability and responsibility in AI include:
- Establishing Lines of Accountability: Clear lines of accountability should be established for AI systems, specifying who is responsible for their development, deployment, and outcomes. This includes identifying the roles and responsibilities of AI developers, deployers, and users.
- Balancing Liability in AI Systems: As AI systems make decisions and take action, questions of liability and responsibility arise. The allocation of liability should be carefully considered, with legal frameworks and regulations addressing the liability implications of AI technologies.
- Ensuring Ethical Responsibility in AI Development and Use: Ethical considerations should be integrated into the design, development, and use of AI systems. This includes incorporating ethical frameworks and guidelines into the development process, conducting ethical impact assessments, and promoting ethical awareness and training among AI developers and users.
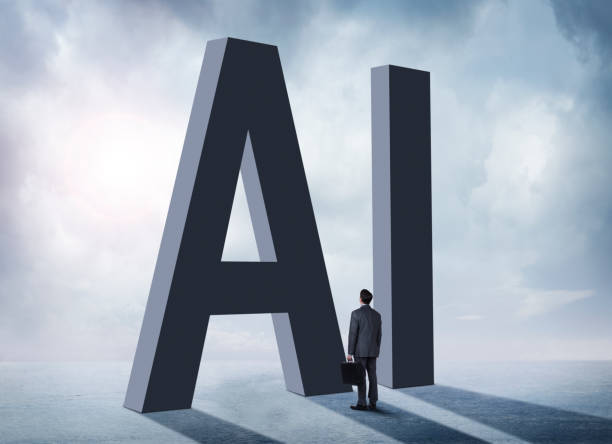
Scaling AI Safely
Scaling AI involves expanding its application across various domains and increasing its impact on individuals, organizations, and society as a whole.
Robust Testing and Validation
Before scaling AI technologies, it is essential to subject them to rigorous testing and validation processes. This helps identify and rectify potential biases, errors, vulnerabilities, or limitations that may arise during their operation. Key aspects of robust testing and validation include:
- The Significance of Testing in AI Development: Comprehensive testing is crucial to assess the performance, accuracy, and reliability of AI systems. This includes conducting unit tests, integration tests, and end-to-end testing to ensure that the AI algorithms and models function as intended.
- Identifying and Addressing Biases and Vulnerabilities: Testing should include evaluating AI systems for potential biases and vulnerabilities. This involves assessing the impact of training data, algorithmic decision-making, and potential sources of bias to identify and mitigate any discriminatory or unfair outcomes.
- Ensuring Safety and Reliability in AI Systems: Testing should focus on verifying the safety and reliability of AI systems. This includes evaluating their robustness, resilience to adversarial attacks, and performance in different scenarios to ensure they function reliably and predictably.
Continuous Monitoring and Evaluation
Once AI systems are deployed at scale, continuous monitoring and evaluation are crucial to address emerging ethical concerns, biases, or issues that may arise during their operation. Continuous monitoring and evaluation help maintain the effectiveness, fairness, and safety of AI technologies. Key aspects of continuous monitoring and evaluation include:
- The Need for Ongoing Monitoring of AI Systems: AI systems should be continuously monitored to ensure they operate as intended and remain aligned with ethical considerations and legal requirements. This includes monitoring for biases, unintended consequences, and changes in the data or operating environment that may affect system performance.
- Addressing Emerging Ethical Concerns: Continuous monitoring enables the identification of emerging ethical concerns and potential biases. Regular assessments should be conducted to address any unintended consequences or unfair outcomes, allowing for timely intervention and corrective measures.
- Regular Audits and Reviews for Ethical Compliance: Independent audits and reviews of AI systems should be conducted to assess their compliance with ethical guidelines, legal requirements, and industry standards. These audits provide transparency, ensure accountability, and help build trust in the deployment and use of AI technologies.
Human-in-the-Loop Approach
Maintaining human involvement and control is crucial for scaling AI safely. The human-in-the-loop approach ensures that human judgment, oversight, and intervention are integrated into AI systems’ operations and decision-making processes. Key aspects of the human-in-the-loop approach include:
- The Importance of Human Oversight in AI Technologies: Human oversight is essential to ensure that AI systems operate within ethical boundaries and legal frameworks. Human involvement allows for the exercise of judgment, consideration of contextual factors, and intervention in critical situations.
- Maintaining Human Control and Intervention: AI systems should be designed to include mechanisms for human control and intervention. This includes establishing decision thresholds, allowing humans to override AI decisions, and enabling human review and validation of AI-generated outputs.
- Balancing Automation with Human Judgment and Ethics: The human-in-the-loop approach ensures a balance between automation and human judgment. AI technologies can automate routine or data-driven tasks, while human judgment and expertise are applied to handle complex or value-laden decisions, ensuring ethical considerations are appropriately addressed.
Gradual Deployment and Iterative Improvements
Scaling AI technologies should be done incrementally, allowing for iterative improvements based on feedback and insights gained from real-world deployment. Gradual deployment helps mitigate potential risks and enables continuous learning and refinement. Key aspects of gradual deployment and iterative improvements include:
- The Benefits of Incremental Scaling: Gradual deployment allows for a controlled and measured expansion of AI technologies. This approach helps identify and address issues or challenges before widespread implementation, reducing the potential for unintended consequences or negative impacts.
- Learning from Real-World Deployment: Real-world deployment provides valuable insights and feedback for improving AI technologies. By monitoring and evaluating AI systems in practical settings, developers can gather data and observations that inform iterative improvements and help enhance system performance and safety.
- Iterative Refinement for Ethical and Safe AI Scaling: Feedback from users, stakeholders, and ethical considerations should inform iterative refinements of AI systems. This includes addressing biases, privacy concerns, and ethical challenges, as well as continuously improving system performance, explainability, and accountability.
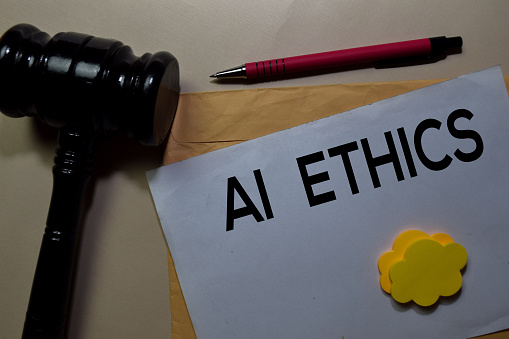
Governance Framework for ChatGPT Usage
AI can potentially bring about tremendous benefits to society, but it also poses ethical and safety challenges. As AI technology becomes more advanced and more widely used, it is important to ensure that AI is developed and used in a responsible and ethical manner. Here are some key considerations for responsible governance and scaling AI safely:
- Transparency: AI systems should be designed and developed in a transparent manner, with clear documentation and communication of the underlying technology and its capabilities.
- Accountability: Developers and users of AI systems should be held accountable for any negative consequences that may arise from their use.
- Fairness and non-discrimination: AI systems should be designed to avoid discrimination and ensure fairness across different demographic groups.
- Privacy and security: AI systems should be designed and developed with appropriate privacy and security measures in place to protect user data and prevent unauthorized access.
- Human oversight: AI systems should be designed to include human oversight and control to ensure that the technology is being used in a responsible and ethical manner.
- Ethical considerations: AI developers and users should consider the broader ethical implications of their work, and ensure that the technology is being used to promote social good.
Conclusion
Ethical responsible governance and safe scaling of AI technologies are essential for harnessing the benefits of AI while upholding human values and societal well-being. Ethical considerations, responsible governance frameworks, and cautious scaling practices ensure fairness, transparency, accountability, and privacy in AI development, deployment, and use. By adopting a proactive approach to ethics and governance, governments, organizations, and developers can mitigate risks, build trust, and foster responsible AI innovation. It is through collaboration, education, and ongoing evaluation that we can ensure that AI technologies are developed and scaled in a manner that aligns with our shared values and contributes positively to society.