Artificial Intelligence (AI) has been at the forefront of technological advancements in recent years, transforming various industries. One area where AI is making a significant impact is in the field of intellectual property (IP) and patent landscape monitoring. In this comprehensive article, we will delve into the world of AI-driven patent landscape monitoring, its importance, applications, challenges, and the tools and platforms that make it possible.
Patent landscape monitoring refers to the process of tracking and analyzing the patent landscape within a specific technological field or industry. This involves the identification and assessment of existing patents, emerging technologies, and the competitive landscape. It plays a pivotal role in guiding business strategies, facilitating innovation, and ensuring that companies protect their intellectual property rights effectively.
The Importance of Patent Landscape Monitoring
Understanding the patent landscape is crucial for businesses and organizations for several reasons:
- Innovation Strategy: It helps in identifying gaps in the market and areas where innovation is needed.
- Competitive Analysis: Provides insights into the IP strategies of competitors.
- Risk Mitigation: Helps in avoiding patent infringements and legal disputes.
- Technology Licensing: Identifies opportunities for licensing and collaboration.
- Market Research: Aids in market analysis and forecasting.
Role of AI in Patent Landscape Monitoring
AI has emerged as a powerful tool in patent landscape monitoring, automating and enhancing the process. AI technologies such as machine learning, natural language processing, image recognition, and graph analysis have revolutionized the way businesses and IP professionals analyze patent data.
This article aims to provide an in-depth exploration of AI’s role in patent landscape monitoring. We will discuss the traditional approaches and their limitations, delve into the various AI applications, examine tools and platforms, address challenges, ethical considerations, and best practices, and explore future trends. Through this, we hope to shed light on the transformation AI brings to the field of intellectual property.
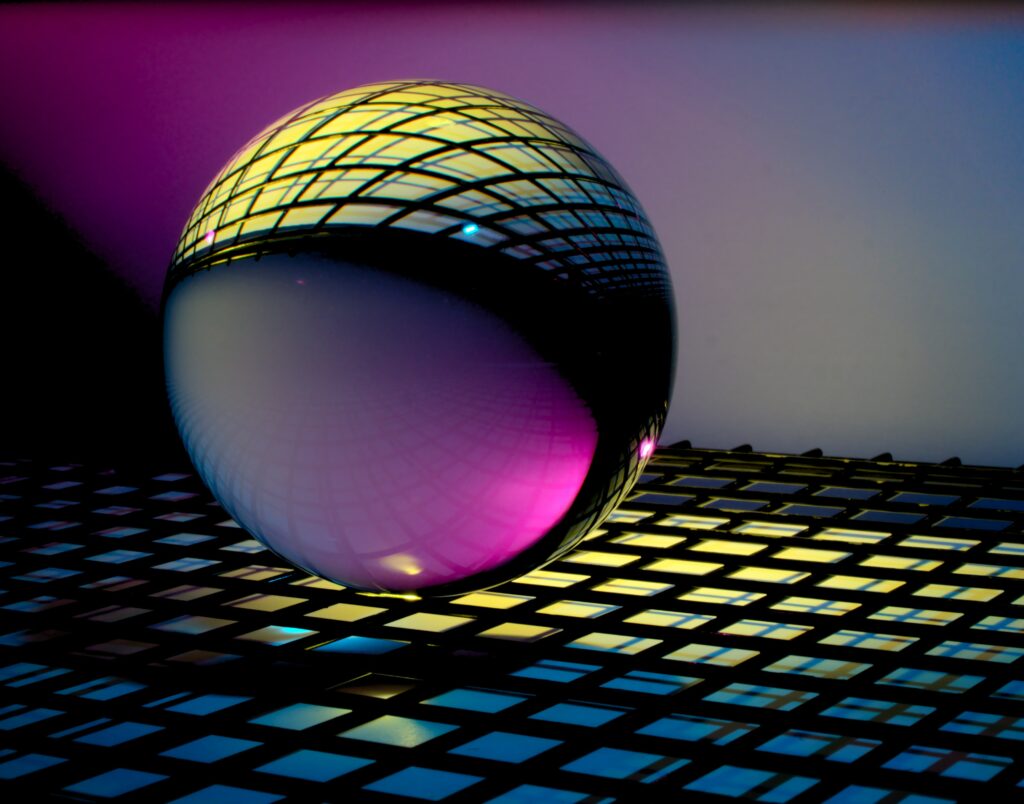
Understanding Patent Landscapes
A patent landscape is a comprehensive view of all patents within a particular technological area. It includes information on existing patents, their ownership, classifications, and citation networks. This data helps in understanding the competitive environment and technological trends.
Key Components of Patent Landscapes
- Patent Documents: These are the core sources of information. They contain detailed descriptions of inventions, legal claims, and drawings.
- Patent Classification: Patents are categorized into classes and subclasses based on their subject matter. This classification aids in organizing and searching for patents.
- Citation Networks: Patents often cite other patents as prior art. Understanding these connections is valuable for identifying influential patents and trends.
The Role of Patent Landscapes in Business and Innovation
Patent landscapes are indispensable for decision-making in various aspects:
- Innovation: Companies can use patent landscapes to identify areas where innovation is needed.
- Competitive Intelligence: Analyzing competitors’ patents provides insights into their technological strengths and weaknesses.
- Legal Compliance: It helps in avoiding patent infringement, which can lead to costly legal battles.
- Investment Opportunities: Patent landscapes can uncover opportunities for investment or partnerships.
Manual Search and Analysis
Historically, patent landscape monitoring was a manual and time-consuming process. Intellectual property professionals had to sift through countless patent documents, categorize them, and draw insights. This approach was not only labor-intensive but also limited in its ability to handle the vast amount of patent data generated today. Limitations of traditional approaches necessitated a shift toward automation. This is where AI comes into play, offering the potential to process and analyze vast amounts of patent data rapidly and accurately.
The Power of AI in Patent Landscape Monitoring
1. Automated Data Processing
One of the most significant contributions of AI to patent landscape monitoring is its ability to process vast amounts of data quickly and accurately. Patent databases contain millions of documents, and manually sifting through them is time-consuming and error-prone. AI, particularly machine learning and natural language processing, can automate data extraction and categorization. It can recognize inventors, assignees, key technical terms, and legal claims, allowing for rapid organization and analysis of patent data.
2. Semantic Search and Context Understanding
AI-driven semantic search is a game-changer in patent landscape monitoring. Unlike traditional keyword-based searches, semantic search understands the context of the query. This means it can recognize synonyms, related terms, and even interpret the user’s intent. For instance, if you’re searching for patents related to “smartphones,” a semantic search can also retrieve documents related to “mobile devices” or “cell phones.” This advanced search capability helps users find highly relevant patents more efficiently.
3. Citation Network Analysis
Understanding the citation networks among patents is vital for recognizing influential patents and technological trends. AI can analyze these networks to identify which patents are frequently cited, revealing important innovations. It helps in understanding the dynamics of technological progress, allowing businesses to stay at the forefront of their industries.
4. Image Recognition
Many patents include drawings, diagrams, and images that describe inventions. AI, through image recognition technology, can extract information from these visuals. This capability expands the scope of patent analysis to include inventions that are primarily described using images. For example, in the field of medical devices, AI can analyze diagrams to identify innovative medical equipment.
5. Predictive Analytics
AI can also make predictions about technology trends and emerging markets. By analyzing historical patent data, machine learning models can identify patterns and forecast where innovation is likely to occur. This predictive capability is immensely valuable for businesses aiming to proactively invest in emerging technologies.
6. Real-time Monitoring
AI has the capacity to offer real-time or near-real-time patent landscape monitoring. This allows organizations to stay ahead of trends, identify potential threats, and seize opportunities as they arise. Keeping pace with rapid technological developments is crucial in competitive industries.
7. Accuracy and Consistency
AI ensures that the data extracted and insights generated are consistent and accurate. It eliminates human errors and inconsistencies that can occur in manual analysis. This accuracy is crucial when making important decisions related to intellectual property, competitive analysis, and innovation strategy.
8. Unbiased Analysis
AI offers a level of objectivity that can be challenging to achieve with human analysts. AI algorithms analyze data based on predefined criteria, reducing the potential for bias. This objectivity is essential in patent landscape monitoring, especially in highly competitive industries.
9. Scalability
AI is highly scalable and can handle large volumes of patent data. This is particularly relevant in today’s information-rich world, where the number of patents being filed is increasing. AI’s scalability ensures that organizations can keep pace with the growing volume of patent information.
10. Integration with Other Technologies
AI can be integrated with emerging technologies like blockchain and quantum computing to enhance data security, traceability, and the ability to solve complex patent analysis problems at unprecedented speeds.
The combination of these capabilities is revolutionizing patent landscape monitoring, making it more efficient, accurate, and insightful. Organizations that embrace AI in this context gain a competitive advantage, improve their innovation strategies, and mitigate risks effectively.
Advantages of AI in Patent Landscape Monitoring
AI brings several advantages to the table:
- Speed and Efficiency: AI can process and analyze vast amounts of data quickly, providing insights in real-time or near-real-time.
- Accuracy: The automation of data extraction and analysis minimizes the risk of human error, leading to more precise results.
- Scalability: AI systems can handle a large number of patents, making them suitable for modern patent landscape monitoring.
- Unbiased Analysis: AI algorithms can provide unbiased analysis by eliminating human subjectivity.
Applications of AI in Patent Landscape Monitoring
Competitive Analysis
AI allows businesses to gain a competitive edge by providing insights into the patent strategies of competitors. This includes understanding the scope and strength of their patent portfolios, the technologies they prioritize, and their innovation trends.
Innovation Strategy
Patent landscapes generated by AI can uncover unexplored areas in a particular technology domain. This data can guide companies in setting innovation strategies and identifying opportunities for new product development.
Technology Licensing and Acquisition
AI-powered patent landscape analysis aids in identifying potential licensing or acquisition targets. Companies can pinpoint technologies that align with their strategic goals and negotiate deals more effectively.
Risk Management
AI can help companies identify potential risks related to patent infringement. By analyzing the patent landscape, businesses can take proactive steps to avoid legal disputes.
IP Portfolio Management
For companies with extensive patent portfolios, AI can streamline the management of intellectual property assets. It can help in categorizing patents, identifying opportunities for monetization, and maintaining IP portfolios efficiently.
Market Research and Forecasting
AI’s ability to process and analyze vast amounts of data enables better market research. Businesses can track technology trends, identify emerging markets, and make data-driven decisions.
Challenges and Ethical Considerations
Data Privacy and Security
One of the foremost challenges in patent landscape monitoring is the handling of vast amounts of sensitive information. Patents often contain detailed descriptions of innovations, which can be valuable to competitors. Protecting this information from unauthorized access and ensuring compliance with data privacy regulations is crucial.
Quality of AI-Generated Insights
AI systems are only as good as the data they are trained on. If the training data is biased or incomplete, the insights generated by AI tools may also be biased or inaccurate. Ensuring the quality and relevance of the data used by AI in patent landscape monitoring is an ongoing challenge.
Intellectual Property and Legal Issues
The use of AI in patent analysis can raise legal questions. For instance, who owns the rights to AI-generated inventions or innovations? Understanding the legal implications and ensuring that AI tools adhere to intellectual property laws is essential.
Bias and Fairness
AI algorithms can inadvertently perpetuate biases present in the data they are trained on. In patent landscape monitoring, this could result in unfair advantages or disadvantages for certain inventors or companies. Ensuring that AI systems are fair and unbiased is a critical ethical consideration.
Transparency and Explainability
AI algorithms, particularly deep learning models, can be seen as “black boxes.” This lack of transparency can make it challenging to understand how AI systems arrive at certain conclusions. In patent landscape monitoring, the ability to explain AI-generated insights is important for trust and accountability.
Addressing these challenges and ethical considerations is essential to ensure the responsible and effective use of AI in the context of patents. Implementing best practices can help navigate these issues.
Best Practices for Implementing AI in Patent Landscape Monitoring
Data Preparation and Cleaning
Thorough data preparation is essential. This includes cleaning and structuring patent data, ensuring it’s accurate and relevant for analysis. Data cleaning involves removing duplicates, standardizing formats, and handling missing information.
Choosing the Right AI Model
Selecting the appropriate AI model is crucial. Different tasks, such as semantic search, image analysis, or citation network analysis, may require different AI approaches. The choice of model should align with the specific goals of the patent landscape monitoring project.
Human-AI Collaboration
AI should augment human expertise, not replace it. Human oversight is critical to interpret AI-generated insights, make informed decisions, and ensure the technology aligns with the organization’s goals and values.
Continuous Learning and Adaptation
AI models need to adapt to changes in the patent landscape. Patents are continually filed and updated. AI systems must be capable of learning and evolving to stay relevant.
In addition to best practices, understanding the future trends and developments in the field of AI for patent landscape monitoring is essential. These trends can guide organizations in adopting the right strategies and tools.
Future Trends and Developments
AI Advancements and Their Impact
As AI technologies continue to advance, we can expect significant improvements in the capabilities of AI-powered patent landscape monitoring tools. These advancements may include:
- Enhanced Accuracy: AI algorithms will become more accurate in understanding patent text and drawing precise insights.
- Expanded Data Sources: Integration with various data sources beyond patent databases, such as scientific literature and news articles, will provide a more comprehensive view of innovation.
- Real-time Monitoring: AI systems will offer real-time monitoring of patent activities, allowing organizations to stay ahead of trends and emerging technologies.
Integration of AI with Emerging Technologies
The synergy between AI and emerging technologies will shape the future of patent landscape monitoring:
- Blockchain: Combining AI with blockchain technology can enhance data security and the provenance of patent information.
- Quantum Computing: Quantum computing holds the potential to solve complex patent analysis problems at unprecedented speeds.
Regulatory Changes and Implications
As AI continues to play a more significant role in patent landscape monitoring, regulatory frameworks are likely to evolve. Companies and organizations need to stay informed about these changes to ensure compliance and ethical use of AI. The future promises exciting developments in the field of AI-driven patent landscape monitoring, with the potential to revolutionize how businesses innovate and protect their intellectual property.