In the intricate realm of patent valuation, where the convergence of academia, innovation, and commerce occurs, leveraging advanced methodologies is paramount. One such methodology making significant strides is data mining. This article delves into the multifaceted role of data mining in patent valuation, uncovering how it transforms the landscape by extracting valuable insights, enhancing accuracy, and shaping the future of valuation practices.
Understanding Data Mining in the Context of Patent Valuation
Before delving into the specifics, it’s crucial to grasp the essence of data mining and its application in the intricate world of patent valuation. Data mining involves extracting patterns, information, and knowledge from large datasets. In the context of patents, this process involves scrutinizing vast repositories of information related to intellectual property to uncover hidden trends, correlations, and potential commercial applications.
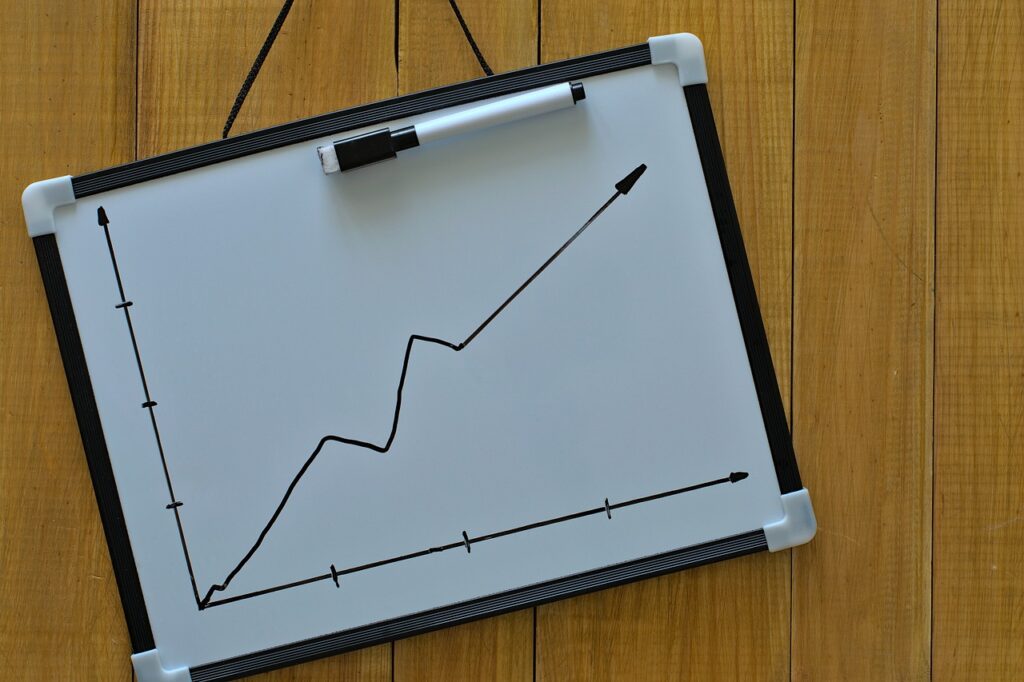
At its core, data mining relies on a combination of statistical algorithms, machine learning techniques, and artificial intelligence to navigate through the vast troves of patent data. By identifying patterns and relationships within the data, it enables patent professionals to make informed decisions regarding the valuation of intellectual property.
The Synergistic Integration of Data Mining and Patent Valuation
The convergence of data mining and patent valuation represents a transformative paradigm shift in the way we assess the worth of intellectual property. This integration goes beyond mere adoption; it signifies a union that brings forth a synergy, fundamentally enriching the valuation process and offering a more profound understanding of the myriad factors that influence the value of a patent.
In the traditional landscape of patent valuation, reliance on historical data and industry benchmarks often forms the crux of assessment methodologies. However, the introduction of data mining introduces a novel dimension. It’s not merely an additional tool; it’s the infusion of a dynamic and data-driven approach that transcends the limitations of conventional valuation methods.
Data mining, with its sophisticated algorithms and machine learning capabilities, delves into vast repositories of patent-related information. This expansive exploration allows for the identification of hidden patterns, correlations, and insights that might elude traditional valuation methods. By extracting these nuances, data mining enriches the valuation process, providing a more comprehensive and detailed perspective on the intrinsic value of a patent.
A Nuanced Understanding of Factors
What sets apart this union is its ability to provide a nuanced understanding of the myriad factors influencing the value of a patent. Traditional methods might focus on historical performance and market trends, but data mining goes deeper. It considers the intricate interplay between diverse variables, offering a more granular and holistic assessment of a patent’s potential applications, market relevance, and overall significance.
Transcending Traditional Boundaries
The marriage of data mining and patent valuation transcends the confines of traditional approaches. It’s not about replacing established methods but augmenting them with a more dynamic and adaptable tool. This synergy allows for a more accurate and forward-looking valuation that aligns with the dynamic nature of innovation, particularly in the academic and industry sectors.
In essence, the integration of data mining into patent valuation is more than a technological advancement; it’s a strategic evolution in how we perceive and quantify the value of intellectual property. By embracing this synergy, stakeholders in the patent ecosystem can navigate the complexities of valuation with greater precision, ensuring a more informed and strategic approach to harnessing the potential of academic and industry patents.
Unveiling the Impact on Accuracy and Predictive Analytics
Enhancing Accuracy Through Pattern Recognition
One of the primary advantages of incorporating data mining in patent valuation is the ability to enhance accuracy through pattern recognition. Traditional valuation methods often rely on historical data and industry benchmarks, but data mining goes beyond this by identifying intricate patterns and trends within the patent data itself. This enables a more precise valuation that considers the unique characteristics and potential applications of each patent.
Predictive Analytics: Peering into the Future
Data mining’s prowess extends to predictive analytics, allowing patent professionals to peer into the future of a technology or innovation. By analyzing historical data and identifying emerging patterns, data mining facilitates the anticipation of future market trends and potential applications. This foresight empowers stakeholders to make strategic decisions, not just based on current market conditions but with a vision of the evolving landscape.
Navigating the Challenges: Quality, Quantity, and Ethical Considerations
The Challenge of Data Quality
In the realm of data mining, a significant challenge lies in ensuring the quality of the underlying data. The success of data mining endeavors hinges on the accuracy and reliability of the insights derived. When applied to patents, this challenge becomes particularly pronounced, emphasizing the importance of comprehensive and precise information within databases. The nuances of patent data, ranging from technical details to legal intricacies, require a meticulous approach to guarantee that the mined insights are meaningful and trustworthy. Without a foundation of high-quality data, the potential of data mining in advancing patent valuation is compromised.
Ensuring the completeness and accuracy of patent information within databases is crucial for meaningful analysis. The multifaceted nature of patents demands a comprehensive dataset that reflects the intricacies of each innovation. Incomplete or inaccurate data can lead to distorted insights, hindering the effectiveness of data mining in uncovering valuable patterns and trends. Addressing the challenge of data quality involves not only refining the collection processes but also establishing rigorous standards for data accuracy, laying the groundwork for more robust and reliable patent valuation through data mining.
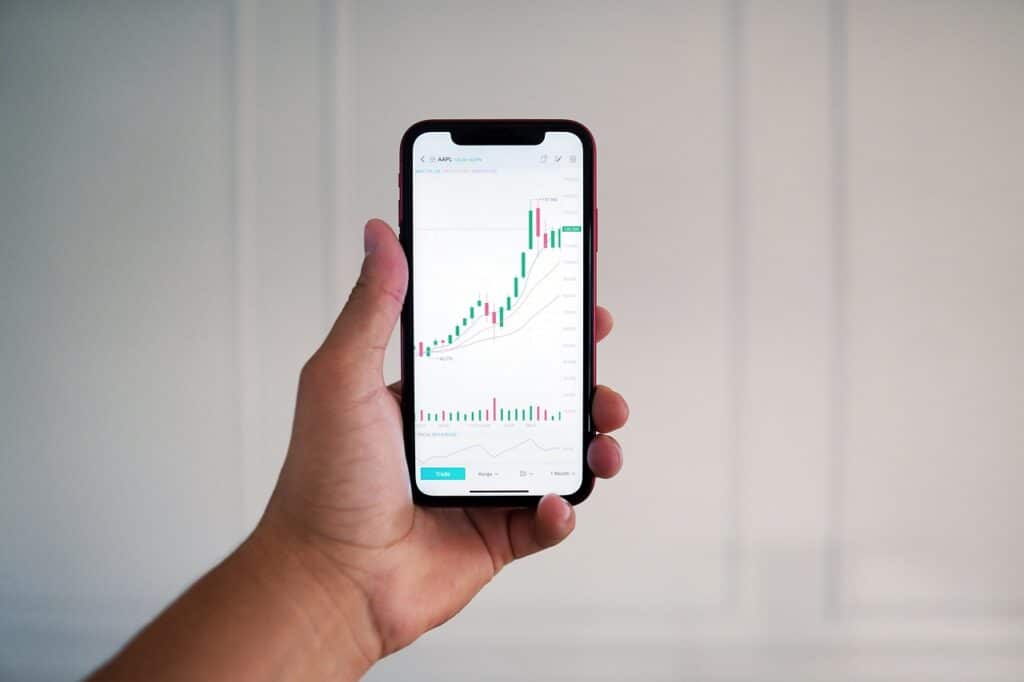
Quantity vs. Quality: Striking a Balance
A delicate balance between the quantity and quality of data is essential in the arena of data mining. The sheer volume of available patent data presents both an opportunity and a challenge. The temptation to prioritize quantity over quality can be compelling, especially when dealing with extensive patent databases. However, a nuanced and strategic approach that values the relevance and accuracy of data over sheer volume is indispensable for extracting meaningful insights.
Striking this balance involves a careful consideration of the research objectives. While a vast dataset may offer a breadth of information, the true value lies in the relevance of the data to the specific goals of patent valuation. A targeted and selective approach ensures that the data used in mining aligns with the intricacies of the valuation process. By prioritizing quality over quantity, stakeholders can avoid the pitfalls of data overload, fostering more meaningful and actionable insights in the complex landscape of patent valuation through data mining.
Ethical Considerations in Patent Data Mining
The exploration of patent data through data mining techniques introduces a host of ethical considerations that demand careful attention. Privacy concerns, potential biases in the data, and the responsible use of information become critical aspects that need to be addressed. Striking a delicate balance between extracting valuable insights and respecting ethical principles is imperative to ensure the integrity of the patent valuation process.
Privacy considerations arise as patent data often involves sensitive information related to individuals, companies, or research institutions. Balancing the need for transparency and the protection of intellectual property with privacy concerns is a delicate task. Additionally, potential biases within the data, whether inherent or introduced through the mining process, must be acknowledged and mitigated to avoid skewed results. Responsible use of information involves ethical decision-making throughout the data mining journey, from data collection to interpretation, safeguarding the ethical foundation of patent valuation practices in an era where data mining plays an increasingly integral role.
Real-World Applications: Case Studies Illustrating Data Mining Successes
Case Study 1: Pharmaceutical Breakthroughs
One striking example of data mining’s impact on pharmaceutical breakthroughs comes from Pfizer’s exploration of patent data. In their quest for novel drug candidates, Pfizer utilized advanced data mining algorithms to sift through extensive patent databases. By analyzing patterns in patents related to successful drug developments, Pfizer researchers identified a promising compound for further investigation. This breakthrough significantly expedited the drug discovery process, allowing Pfizer to bring a potential life-saving medication to market more efficiently. The valuation of the associated patent was bolstered by the precise insights gleaned through data mining, showcasing how this approach directly translates into tangible advancements in pharmaceutical innovation.
Case Study 2: Technological Convergence
In the realm of technological convergence, a noteworthy case involves IBM’s strategic use of data mining to assess patents at the intersection of artificial intelligence and healthcare. IBM’s data mining techniques, applied to a vast array of patents in these fields, revealed groundbreaking synergies. Specifically, the algorithms identified patents that seamlessly integrated AI technologies into healthcare applications, foreseeing a transformative impact on patient care. This case exemplifies how data mining’s ability to uncover hidden connections between disparate technological domains can lead to a more nuanced and accurate valuation of patents, influencing strategic decisions in the dynamic landscape of healthcare innovation.
These real-life specific cases vividly demonstrate the practical applications of data mining in pharmaceutical breakthroughs and technological convergence, showcasing how this powerful tool is instrumental in identifying valuable patents and shaping the trajectory of innovation in diverse industries.
The Future Trajectory: Data Mining in the Evolving Landscape of Patent Valuation
Integration with Blockchain for Enhanced Security
Looking ahead, the convergence of data mining and blockchain technology holds significant promise in fortifying the security and integrity of patent data. Blockchain’s inherent attributes of decentralization and tamper-resistant ledgers provide an ideal framework for safeguarding the sanctity of patent information. The integration of data mining with blockchain could be a game-changer, ensuring that the insights derived from data mining remain unaltered and trustworthy in the ever-evolving landscape of patent valuation.
The decentralized nature of blockchain aligns seamlessly with the need to protect patent data from unauthorized tampering. Each block in the chain contains a unique cryptographic hash, making it resistant to alteration. This level of security ensures that the data mined for patent valuation retains its original integrity, instilling confidence in the accuracy of assessments. The immutable and transparent nature of blockchain technology addresses the growing concerns regarding data security, positioning it as a robust solution for the future of patent valuation.
AI-Powered Sentiment Analysis for Market Perception
In the future trajectory of data mining in patent valuation, a notable evolution involves the integration of sentiment analysis powered by artificial intelligence (AI). This innovative approach goes beyond the quantitative insights derived from data mining by introducing a qualitative layer focused on market perceptions and sentiments surrounding a patent or technology.
By leveraging AI-powered sentiment analysis, stakeholders can gain valuable insights into how a patent is perceived in the market. Understanding the qualitative aspects of market reception goes beyond the raw data, offering a more holistic view of a patent’s potential impact. Positive or negative sentiments can significantly influence the perceived value of a patent, influencing investment decisions, licensing agreements, and overall market positioning. This integration represents a nuanced step forward, acknowledging the importance of qualitative factors in the complex landscape of patent valuation.
Continued Emphasis on Ethical Data Mining Practices
As data mining continues its evolution, a consistent and unwavering emphasis on ethical practices remains paramount. Stakeholders engaged in patent valuation must prioritize transparency, fairness, and responsible use of data. The ethical considerations surrounding data mining in the context of patents extend to privacy concerns, potential biases, and the overall impact on society.
Ethical guidelines and frameworks for data mining in patent valuation are indispensable. Establishing and adhering to ethical practices ensure that this powerful tool is wielded responsibly for the benefit of innovation and society. As data mining becomes more integral to the patent valuation process, maintaining ethical standards becomes a cornerstone, fostering trust, credibility, and the sustainable advancement of the field.
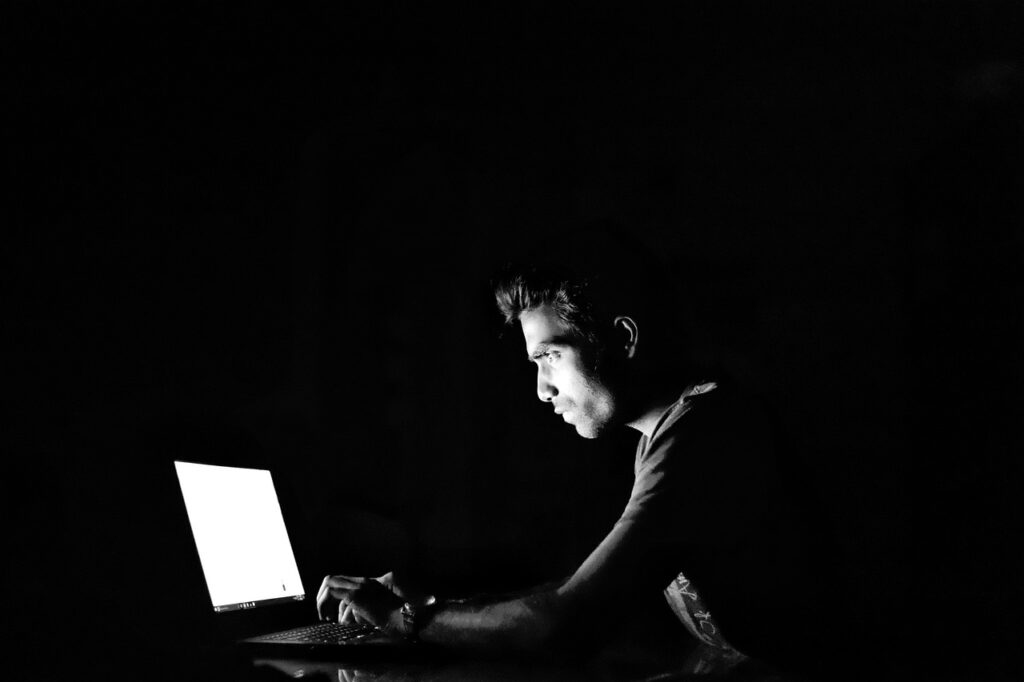
Conclusion
In conclusion, the integration of data mining in patent valuation represents a transformative shift in how we approach the assessment of intellectual property. From enhancing accuracy and predictive analytics to navigating challenges and real-world applications, data mining emerges as a powerful ally in the valuation landscape. As we look to the future, the trajectory of data mining in patent valuation holds the promise of continued innovation, ethical considerations, and a more comprehensive understanding of the value inherent in the patents that shape our technological landscape.