When it comes to personal injury cases, the complexities can be overwhelming. From gathering evidence to assessing liability and estimating damages, every detail matters. Traditionally, lawyers and legal professionals have relied on their experience and legal expertise to navigate these intricate waters. However, in recent years, a powerful ally has emerged to assist in personal injury case assessment: machine learning. In this comprehensive guide, we’ll delve into the world of machine learning and its application in personal injury cases. We’ll explore how it can revolutionize the way attorneys and insurance companies approach case assessment and litigation.
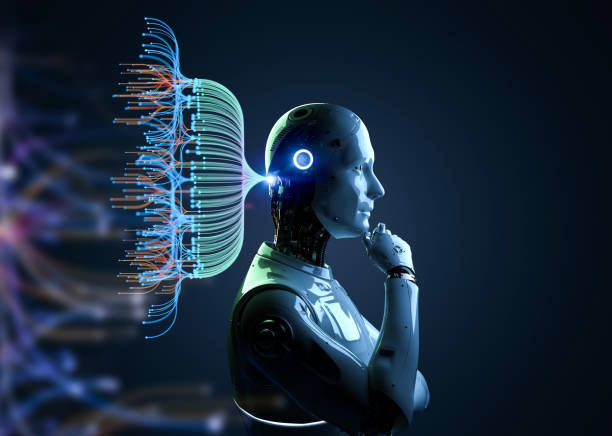
Understanding Machine Learning
Before we dive into its role in personal injury cases, let’s get a solid grasp of what machine learning is all about.
What is Machine Learning?
At its core, machine learning is a subset of artificial intelligence (AI) that focuses on creating algorithms and models that allow computers to learn from and make predictions or decisions based on data. Instead of being explicitly programmed to perform a specific task, machine learning algorithms are designed to improve their performance over time through exposure to data. This capacity for learning and adaptation is what makes machine learning so powerful.
Types of Machine Learning
There are several types of machine learning algorithms, each suited for specific tasks. The three primary categories are:
Supervised Learning
Supervised learning involves training a model on a labeled dataset, where the algorithm learns to make predictions by mapping input data to output labels. It’s widely used in tasks like classification and regression, making it relevant to personal injury case assessment.
Unsupervised Learning
Unsupervised learning algorithms work with unlabeled data, aiming to find patterns or group similar data points together. While less commonly used in personal injury cases, it has potential applications in data exploration.
Reinforcement Learning
Reinforcement learning is about training agents to make decisions in an environment to maximize rewards. While not a primary focus in personal injury cases, it’s an exciting field within machine learning.
Now that we have a foundational understanding of machine learning, let’s explore how it can be harnessed in personal injury case assessment.
The Role of Machine Learning in Personal Injury Cases
Machine learning has the potential to transform the legal landscape, particularly in personal injury cases. Its ability to process vast amounts of data, identify patterns, and make predictions can significantly enhance the efficiency and accuracy of case assessment. Here’s a closer look at how it can be applied:
Data Collection and Analysis
One of the most time-consuming aspects of personal injury case assessment is collecting and analyzing relevant data. Machine learning algorithms can streamline this process by automatically extracting data from various sources, such as medical records, accident reports, and witness statements. This not only saves valuable time but also reduces the risk of overlooking critical information.
Liability Assessment
Determining liability is often a complex task that involves assessing the actions of all parties involved in an accident. Machine learning can help by analyzing historical case data and identifying trends and factors that contribute to liability. For example, it can assess factors like traffic conditions, weather, and driver behavior to determine the likelihood of liability for a specific case.
Estimating Damages
Calculating damages in a personal injury case requires a thorough evaluation of medical bills, lost wages, pain and suffering, and other relevant factors. Machine learning models can assist in this process by analyzing historical settlement data and identifying patterns that influence the final settlement amount. This can provide attorneys and insurance adjusters with more accurate estimates and negotiation strategies.
Predicting Case Outcomes
Machine learning excels at making predictions based on historical data. In personal injury cases, it can be used to predict the likely outcome of a case based on factors such as the jurisdiction, judge, and the specifics of the accident. This predictive capability can help attorneys and clients make informed decisions about whether to settle or proceed to trial.
Challenges and Considerations
While machine learning offers significant potential in personal injury case assessment, it’s important to acknowledge the challenges and considerations associated with its implementation.
Data Privacy and Security
Personal injury cases involve sensitive and confidential information, including medical records and financial data. Ensuring the privacy and security of this data is paramount when implementing machine learning solutions. Legal professionals must carefully select and vet technology partners with robust data protection measures in place.
Model Interpretability
Machine learning models can be complex, making it challenging to interpret their decisions. In legal contexts, it’s crucial to have transparent and interpretable models to justify case assessments and predictions. Striking a balance between model accuracy and interpretability is essential.
Ethical Considerations
The use of machine learning in personal injury cases raises ethical questions about bias and fairness. Models trained on historical data may inherit biases present in that data. Legal professionals must actively address these biases and ensure that assessments and decisions are fair and unbiased.
Human Expertise
Machine learning should complement, not replace, human expertise. While algorithms can analyze data and make predictions, the nuanced understanding of legal intricacies and negotiation skills remain vital. Legal professionals should view machine learning as a tool to enhance their capabilities, not a replacement for their expertise.
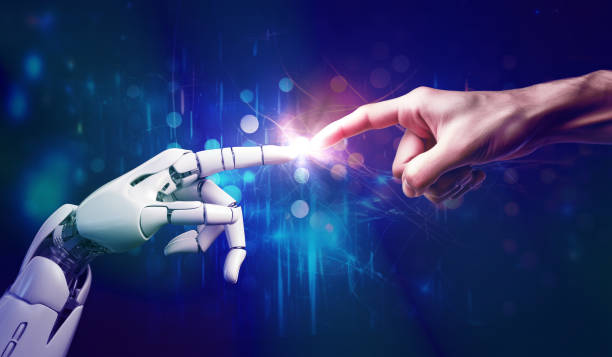
Case Studies: Real-World Applications
To better understand the practical implications of machine learning in personal injury case assessment, let’s explore a few real-world case studies.
Case Study 1: Predicting Settlement Amounts
A law firm specializing in personal injury cases implemented a machine learning model to predict settlement amounts based on historical case data. The model analyzed factors such as injury severity, medical expenses, and jurisdiction. By using this predictive tool, the firm achieved more accurate estimates of settlement amounts, allowing clients to make informed decisions about accepting offers or pursuing litigation.
Case Study 2: Liability Assessment
An insurance company integrated machine learning algorithms into their claims processing workflow. These algorithms assessed factors like weather conditions, accident location, and driver behavior to determine liability in auto accident cases. This streamlined process reduced claim processing time and improved the accuracy of liability assessments.
Case Study 3: Document Review Automation
A legal department within a corporation implemented machine learning to automate the review of medical records and other documents related to personal injury cases. The system extracted relevant information, flagged critical details, and organized the data for attorneys. This automation significantly reduced the time required for document review, allowing legal teams to focus on strategic case planning.
The Future of Personal Injury Case Assessment
As technology continues to advance, machine learning’s role in personal injury case assessment will likely expand. With improved algorithms, enhanced interpretability, and a focus on ethical considerations, the legal industry stands to benefit greatly from this powerful tool. However, it’s essential to approach machine learning implementation thoughtfully, recognizing both its potential and its limitations.
The future of personal injury case assessment is not about replacing human expertise but about augmenting it with the capabilities of machine learning. Legal professionals who embrace this synergy can expect more efficient case assessments, more accurate predictions, and ultimately, better outcomes for their clients.
Intrigued by the possibilities of machine learning in personal injury cases? Would you like to delve deeper into specific aspects or explore more case studies? If so, let’s continue our journey into this exciting field of legal technology.
Ethical Considerations in Machine Learning
Before we proceed further into the practical applications of machine learning in personal injury cases, it’s essential to delve into the ethical considerations that underpin this technology’s use in the legal field.
Fairness and Bias
One of the most critical ethical concerns in machine learning is fairness and bias. Models trained on historical data may inadvertently perpetuate biases present in that data. In personal injury cases, this could lead to unfair assessments or discrimination. To mitigate this, legal professionals must actively work to identify and rectify biases in their machine learning models. Regular auditing and adjustment of algorithms are essential steps to ensure fairness.
Transparency and Accountability
Machine learning models can be complex and difficult to interpret. This opacity can raise questions about accountability when automated systems make decisions that affect individuals’ lives. Legal professionals must prioritize transparency, striving to make the decision-making processes of their machine learning models understandable and justifiable.
Data Privacy and Security (Continued)
The ethical responsibility to protect sensitive data extends beyond mere compliance with legal regulations. Legal professionals must go the extra mile to safeguard the privacy and security of personal information. This includes encrypting data, implementing strict access controls, and regularly auditing data handling practices.
Informed Consent
When using machine learning for personal injury case assessment, obtaining informed consent from clients is crucial. Clients have the right to understand how their data will be used and the potential implications of automated assessments. Legal professionals must be transparent about the role of machine learning in their cases and obtain explicit consent from clients.
Advancements in Machine Learning for Personal Injury Cases
The field of machine learning is continually evolving, and innovations are emerging that promise to further enhance its application in personal injury case assessment.
Natural Language Processing (NLP)
Natural Language Processing, a subset of machine learning, focuses on enabling computers to understand, interpret, and generate human language. In personal injury cases, NLP can be employed to analyze vast amounts of text data, such as medical records, witness statements, and legal documents. This can streamline the process of extracting valuable information, improving the speed and accuracy of case assessment.
Explainable AI (XAI)
Explainable AI aims to make machine learning models more transparent and interpretable. In personal injury cases, where transparency is crucial, XAI can help legal professionals understand how a model arrived at a particular decision. This not only improves accountability but also enhances trust in the technology’s assessments.
Predictive Analytics
Predictive analytics is an area of machine learning that focuses on forecasting future outcomes based on historical data. In personal injury cases, predictive analytics can be used to estimate the likelihood of various case outcomes, helping attorneys and clients make informed decisions about case strategies and settlement negotiations.
Enhanced Data Security
With the growing importance of data privacy and security, machine learning tools are continually being developed to strengthen data protection measures. These advancements will enable legal professionals to harness the power of machine learning while ensuring the confidentiality and integrity of sensitive case-related information.
Legal and Regulatory Landscape
The legal and regulatory landscape surrounding machine learning in personal injury cases is evolving to address the ethical and legal implications of this technology.
Legal Standards and Guidelines
Legal bodies are working to establish standards and guidelines for the use of machine learning in the legal field. This includes defining best practices for ensuring fairness, transparency, and accountability when employing machine learning algorithms in personal injury case assessment.
Data Privacy Regulations
The introduction of stringent data privacy regulations, such as the General Data Protection Regulation (GDPR) and the California Consumer Privacy Act (CCPA), has significant implications for how personal data is handled in machine learning applications. Legal professionals must stay up-to-date with these regulations to ensure compliance.
Ethical Frameworks
Legal organizations and institutions are developing ethical frameworks that guide the responsible use of machine learning in personal injury cases. These frameworks emphasize the importance of ethical considerations, including fairness, transparency, and informed consent.
The Human-Machine Partnership
Machine learning is a powerful tool, but it is not a replacement for the expertise and judgment of legal professionals. Instead, it complements their skills, making the process of personal injury case assessment more efficient and accurate. The human-machine partnership in the legal field is a symbiotic relationship that holds great promise for the future.
Leveraging Machine Learning Expertise
Legal professionals should leverage the expertise of data scientists and machine learning specialists to implement and maintain machine learning solutions effectively. Collaborative efforts ensure that the technology aligns with legal objectives and ethical standards.
Continuous Learning and Adaptation
Machine learning models are not static; they require continuous learning and adaptation. Legal professionals must stay engaged with their machine learning systems, regularly monitoring and refining them to ensure their effectiveness and ethical compliance.
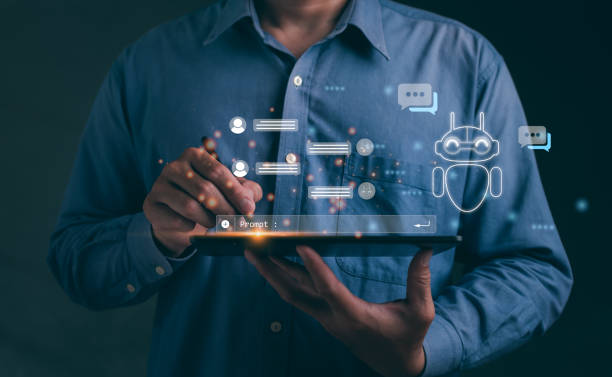
Conclusion
Machine learning in personal injury case assessment represents a significant advancement in the legal field. While it offers the potential for increased efficiency, accuracy, and data-driven insights, it also comes with ethical considerations and the need for responsible implementation. The legal industry is at the cusp of a transformative era, where human expertise and machine capabilities converge to deliver better outcomes for clients and ensure the fair and just handling of personal injury cases.