M&A is a high-stakes, complex arena in which companies make strategic choices to grow, diversify, or gain an edge. The quality of the analysis conducted during due diligence, and after merger integration is often what determines whether an M&A deal will be successful.
Artificial intelligence (AI), and machine learning, (ML), have become indispensable tools for this process in recent years. This essay examines the many ways that AI and ML can contribute to M&A analyses, from automating data processing to enhancing decision-making, and, ultimately, fostering successful M&A results.
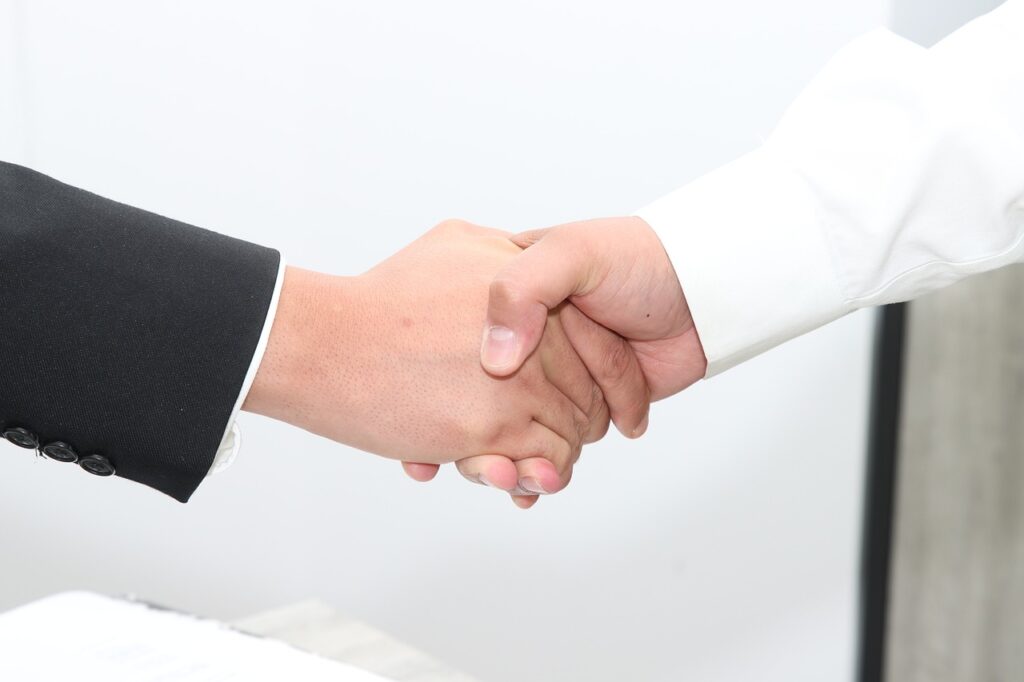
How does AI help in Mergers and Acquisitions Analysis?
Machine learning is a powerful tool that can help analyze mergers and purchases (M&As) in a variety of ways. M&A deals involve many complex decisions in terms of financial, operational, and strategic aspects. ML can be used to assist with several aspects:
Due Diligence
ML automates the extraction and process of large volumes of documents, such as financial statements, legal agreements, and regulatory submissions. Natural Language Processing techniques (NLP) can be used to achieve this. ML can identify risks and opportunities in an M&A transaction by analyzing historical financial data, trends, and benchmarks.
Valuation
M&A analysis requires the identification of business patterns and trends that can help predict future outcomes. This is a tedious and time-consuming process for data scientists. However, predictive modeling solutions are able to automate this task and eliminate the need for advanced technical degrees or dedicated data science teams. Predictive modeling software can also be used to create a model for the current state of the target company and identify possible risks associated with the deal.
Predicting customer churn
Another use for machine learning in M&A is predicting customer churn. Many companies lose customers following M&A deals, and identifying patterns and indicators of churn can help prevent it. This type of analysis is essential to ensure the success of any merger or acquisition.
Machine learning can be used to identify fraudulent activity during M&A transactions. Using the financial and operational data from the two companies involved, machine learning can identify suspicious patterns or anomalies that may indicate fraud. This can provide valuable information to M&A team members that can be used at the negotiating table.
Cultural compatibility
It can be used to assess cultural compatibility between two companies. This can be a vital aspect of M&A and ensure that the companies will be able to work well together. Using a combination of text mining, Natural Language Processing and predictive models, machine learning can analyze the culture of a company to determine whether it will be compatible with the culture of another.
Currently, due diligence takes months, but AI-enabled software applications could reduce this to minutes. This would make M&A faster, less expensive and more efficient. Moreover, these tools can help M&A teams identify the best targets and execute their deals more effectively. ML Models can simulate different scenarios to determine how market conditions or financial variables might affect the valuation of a merged entity.
Competitive Analysis and Market Analysis
Companies need to know the dynamics of their market and the competitive landscape before they engage in M&A transactions. Machine learning algorithms are useful for market segmentation. They can help identify niches or customer bases that could be targeted after a merger. By analyzing data such as customer purchases, market trends and purchase histories, ML models provide insight into the areas where a merged entity could capture more market share.
AI and ML are also excellent at competitor analysis. These technologies can monitor and analyze competitor performance, customer sentiment and market share. ML models are able to provide valuable insight into the strategies, strengths and weaknesses of competitors by leveraging data sources such as news articles, social media and financial reports. This information helps inform the acquisition strategy and identify opportunities for competitive advantages.
Post-Merger Integration
Integration is key to the success of a merger and acquisition. AI and ML are able to optimize resource allocation, which can help with post-merger integration. They can, for example, analyze workforce data in order to determine the most effective distribution of human resources, which will ensure that the correct people are placed in the appropriate roles within the merged organization. This optimization reduces redundancy and costs while maximizing productivity.
AI can also be used to assess cultural and employee fit. By analyzing corporate culture, performance metrics, and work preferences, ML models are able to identify cultural mismatches. They can then provide recommendations for how to minimize them. This helps to maintain employee morale and can make the transition easier.
Regulatory Compliance
Compliance with regulatory requirements is an important concern in the M&A world. It can be difficult to keep up with the changes in laws and regulations that could affect a deal. AI and ML are able to assist with regulatory monitoring, by automatically tracking and assessing changes in laws and regulations that may have an impact on a M&A deal. This proactive approach ensures the parties involved are aware of any compliance requirements, and that they can take action promptly to resolve any issues.
Portfolio Management
After an M&A deal, companies are often required to optimize the portfolio of assets and subsidiary. AI and ML are able to help with this task, by analyzing each asset’s performance and recommending strategies that maximize returns and minimize risk. ML algorithms, for example, can evaluate the financial health, potential growth, and strategic fit each subsidiary. This helps companies decide whether or not to divest from, further invest in, or merge entities.
Analysis of Customer and Supplier Data:
In the post-merger period, maintaining and expanding customer relations is an important consideration. The use of ML to predict customer churn allows companies to identify high-risk customers, and develop retention strategies. By analyzing data such as purchase histories, engagement patterns and customer data, ML models provide insight into customer behavior. This helps the merged entity to tailor its offerings and increase customer satisfaction.
AI and ML are also able to assess the risks of suppliers and, if needed, recommend alternatives. These technologies help companies reduce their dependency on high-risk vendors by analyzing data about suppliers, their financial stability, and performance metrics. It can also predict the financial performances of the merged entity in different scenarios and help stakeholders make informed decisions.
Deal Sourcing
AI and ML are also useful in identifying target companies that can be acquired at the beginning stages of M&A analyses. Data analysis and machine-learning algorithms allow companies to screen and evaluate many potential targets according to financial and strategic criteria. This automated screening process streamlines deal sourcing, allowing organizations to focus on the most promising deals.
Fraud Detection
Financial irregularities and fraudulent activity can have serious consequences in the M&A world. AI and ML are able to detect anomalies in the due diligence phase. These technologies analyze financial data, transaction records, and any other relevant information in order to detect suspicious patterns or discrepancies. Early detection of fraud helps reduce risks and ensures the M&A deal is based on accurate information.
Limitations and considerations
It is important to consider privacy and ethical issues when using AI or ML in M&A analyses. Here are a few key points to keep in mind:
- Data quality: AI models and machine learning models heavily depend on data. Data that is inaccurate or biased can lead to flawed analyses and decisions.
- Interpretability Some machine-learning models are complex and difficult to interpret. Transparency in M&A analyses is essential to gain the trust of stakeholders.
- Human expertise: AI should not replace human expertise, but rather complement it. The human element remains crucial for strategic thinking
Text Mining
An important part of the M&A process is gathering information and data that will ultimately inform the decision to make or pass on a deal. Often, this data comes from different written resources like documents and emails. Machine learning algorithms are well-equipped to sort through this information to find similarities and associations that would be difficult for humans to notice, particularly if the data is large or complex. This kind of analysis can be used to quickly identify potential risks in a deal, as well as help find value-creating opportunities.
One example of this is utilizing machine learning to automate the processes carried out by analysts in the early stages of M&A, such as researching and reaching out to businesses that may be suitable targets for acquisition. This technology can greatly accelerate and increase the scale of this work, allowing analysts to go deeper into areas like a target’s financial performance, sectoral trends and potential post-deal synergies.
Similarly, AI can help M&A teams analyze the rich underlying data that exists alongside pro forma results in order to better understand unique phenomena such as seasonality deviations or outliers in growth that might need further attention and investigation. This kind of deeper analytics can lead to a more informed and confident M&A strategy, providing greater confidence in the process and outcomes.
The M&A market is rapidly evolving and as a result, so are the technologies that support it. Using intelligent automation to streamline key activities is increasingly common in the M&A industry. However, for some firms, implementing these tools can be challenging and costly. This is especially true for smaller or mid-sized companies that do not have the internal capabilities to build their own AI systems, nor the budget to outsource this work.
In the past, introducing AI into an M&A workflow would have required a sophisticated rule-based system that could learn from and analyze a dataset. Today, however, many ML technologies can be used to automate simple and repetitive tasks and significantly reduce the time that would otherwise be spent on them. For example, an algorithm trained on a supervised dataset can be taught to quickly scan and upload files to a virtual data room ahead of due diligence, cutting a task that usually takes months down to