In recent years, machine learning has made remarkable strides in various domains, and its applications in the legal sector have been no exception. Legal AI, powered by machine learning algorithms, has become a valuable tool for lawyers and organizations to streamline legal practice and improve decision-making processes. One critical area where machine learning has demonstrated significant potential is in analyzing employment discrimination cases. In this article, we will delve into the intricate world of Machine Learning for Employment Discrimination Analysis, exploring its applications, benefits, challenges, and the legal implications surrounding its use.
Understanding the Role of Machine Learning in Legal AI
The Intersection of Legal Practice and Machine Learning
Legal practice has historically been a labor-intensive field, requiring attorneys and legal professionals to sift through vast amounts of documents, precedents, and case law to make informed decisions. Machine learning, with its ability to process large datasets and identify patterns, has revolutionized how legal professionals approach their work.
Legal AI powered by machine learning can assist lawyers in numerous ways, from document review and contract analysis to predictive analytics. In the context of employment discrimination analysis, machine learning algorithms can help legal professionals identify hidden biases, uncover discriminatory practices, and make data-driven decisions that can be crucial in litigation.
The Benefits of Using Machine Learning for Employment Discrimination Analysis
Enhanced Efficiency
One of the primary benefits of employing machine learning in employment discrimination analysis is the significant boost in efficiency. Traditional methods of reviewing documents and identifying potential discrimination cases can be time-consuming and error-prone. Machine learning algorithms can quickly scan through thousands of documents, extracting relevant information and highlighting potential issues.
This efficiency not only saves time but also allows legal professionals to focus on higher-level tasks, such as strategizing and building strong cases, rather than getting lost in the paperwork. Additionally, it helps in reducing the backlog of cases, ensuring that justice is served more promptly.
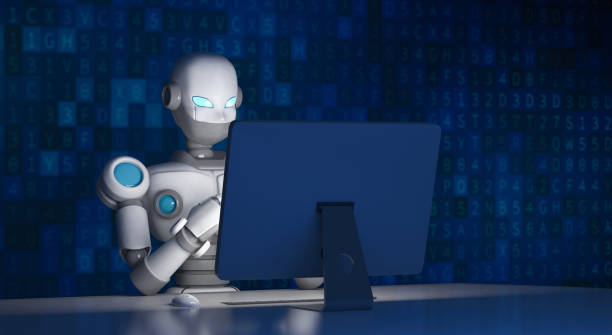
Improved Accuracy
Machine learning algorithms are trained to recognize patterns and anomalies in data, making them invaluable in detecting subtle signs of employment discrimination. They can analyze historical data, employee records, performance reviews, and other relevant information to identify potential discrimination cases that may have been overlooked by human reviewers.
The increased accuracy in identifying discrimination cases can lead to fairer outcomes for employees and employers alike. By leveraging machine learning, legal professionals can ensure that no stone is left unturned when investigating potential instances of discrimination.
Data-Driven Insights
Machine learning doesn’t just stop at identifying potential discrimination cases; it also provides valuable insights through data analysis. By examining patterns in discrimination cases over time, legal AI can help organizations pinpoint areas where discrimination is more likely to occur, allowing for proactive measures to be taken.
For example, a company may discover that a particular department consistently faces higher discrimination complaints. Armed with this knowledge, they can implement targeted diversity and inclusion initiatives and provide additional training to employees in that department.
Overcoming Challenges in Employment Discrimination Analysis
While machine learning offers significant advantages in employment discrimination analysis, it is not without its challenges. Understanding and addressing these challenges is essential to harnessing the full potential of this technology.
Data Bias
One of the primary concerns in employing machine learning for employment discrimination analysis is the presence of bias in the training data. If the training dataset used to develop machine learning models contains biased or incomplete information, the models themselves can perpetuate these biases. This could result in unfair or inaccurate assessments of discrimination cases.
To mitigate data bias, it’s crucial to curate diverse and representative datasets, ensuring that the models are trained on a broad spectrum of cases and scenarios. Additionally, continuous monitoring and auditing of the models can help identify and rectify any bias that may emerge during their use.
Interpretability and Accountability
Machine learning models, particularly deep learning models, can be complex and challenging to interpret. This lack of interpretability can pose a problem in the legal context, where transparency and accountability are paramount.
Legal professionals must be able to explain and justify the decisions made by machine learning models, especially when they have significant implications for individuals or organizations. Developing methods for model interpretability and ensuring that the decisions made align with legal standards are ongoing challenges in the field.
Ethical Considerations
As with any technology, the use of machine learning in employment discrimination analysis raises ethical questions. It’s essential to consider the potential consequences of automated decision-making and the impact it may have on individuals and society as a whole.
For example, relying solely on machine learning models to assess discrimination cases without human oversight may lead to unjust outcomes. Striking the right balance between automation and human judgment is crucial to ensure fairness and accountability in the legal process.
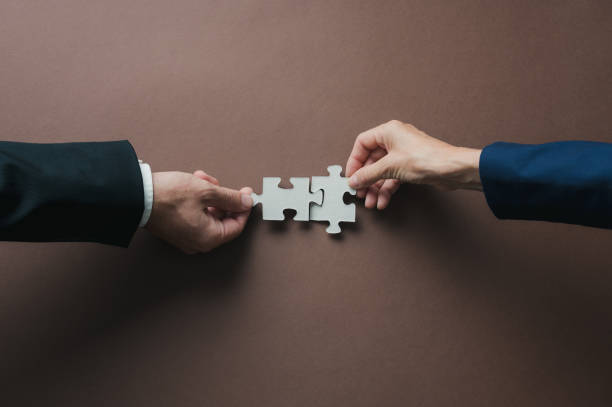
The Legal Implications of Machine Learning in Employment Discrimination Analysis
The use of machine learning in employment discrimination analysis has legal implications that must be carefully navigated. These implications touch upon various aspects of the legal system, including privacy, discrimination laws, and the role of legal professionals.
Privacy Concerns
The collection and analysis of vast amounts of data, often including personal information, raise concerns about privacy. Individuals have a right to privacy, and the use of their data for discrimination analysis must comply with relevant privacy laws and regulations.
Legal AI should be designed to protect sensitive information and ensure that data is handled securely and in accordance with legal requirements. Clear policies and procedures should be in place to address data privacy concerns and gain the trust of individuals involved in discrimination cases.
Compliance with Discrimination Laws
Employment discrimination analysis is closely tied to discrimination laws and regulations. Machine learning models used in this context must adhere to these laws and provide fair and unbiased assessments.
Legal professionals and organizations must ensure that the use of machine learning in discrimination analysis does not result in discriminatory practices themselves. Compliance with existing laws is crucial to avoid legal repercussions.
The Role of Legal Professionals
While machine learning can enhance efficiency and accuracy in employment discrimination analysis, it should complement rather than replace legal professionals. The human element, including legal judgment, ethical considerations, and empathy, plays a crucial role in ensuring fair outcomes in discrimination cases.
Legal AI should be viewed as a tool to assist legal professionals in their work rather than a replacement for their expertise. Lawyers should remain actively involved in the decision-making process and exercise their judgment based on legal principles and ethical considerations.
Specific Machine Learning Algorithms for Employment Discrimination Analysis
To delve deeper into the realm of machine learning for employment discrimination analysis, it’s essential to explore the specific algorithms and techniques employed in this field. These algorithms play a pivotal role in uncovering patterns and making predictions related to discrimination cases.
Logistic Regression
Logistic regression is a commonly used machine learning algorithm in the context of employment discrimination analysis. It’s a type of supervised learning algorithm that is well-suited for binary classification tasks, such as determining whether a discrimination case is likely to be valid or not.
In this approach, historical discrimination cases are used to train the model. The algorithm learns the relationship between various features (e.g., demographic information, employment history) and the binary outcome (discrimination or no discrimination). Once trained, the model can predict the likelihood of discrimination for new cases based on their features.
Logistic regression is valued for its simplicity and interpretability. Legal professionals can examine the coefficients of the model to understand which factors contribute most significantly to discrimination predictions. However, it may not capture complex, non-linear relationships in the data, which is a limitation in cases where discrimination may manifest in subtle ways.
Decision Trees and Random Forests
Decision trees and random forests are versatile machine learning algorithms used in employment discrimination analysis. Decision trees, in particular, are known for their ability to represent complex decision-making processes in a visually intuitive manner.
A decision tree splits the data into subsets based on the values of input features, making binary decisions at each node. These splits are determined by maximizing the separation of discrimination and non-discrimination cases. Decision trees are relatively interpretable, allowing legal professionals to trace the decision path and understand which features contribute to the final outcome.
Random forests, on the other hand, are an ensemble technique that combines multiple decision trees to improve accuracy and reduce overfitting. They are particularly useful when dealing with noisy or complex datasets. Random forests provide feature importance scores, aiding in the identification of discriminatory factors.
Support Vector Machines (SVM)
Support Vector Machines (SVM) are another valuable tool in employment discrimination analysis. SVMs are effective in finding a hyperplane that best separates discrimination and non-discrimination cases in high-dimensional feature spaces.
The key idea behind SVMs is to find the hyperplane that maximizes the margin between the two classes while minimizing misclassifications. This margin represents the confidence of the model’s predictions. SVMs are especially useful when dealing with cases where the discrimination boundary is not linear but can be nonlinearly transformed into a higher-dimensional space.
However, SVMs may require careful parameter tuning, and their interpretation can be challenging in high-dimensional spaces. Legal professionals often work closely with data scientists to ensure the appropriate use of SVMs in discrimination analysis.
Neural Networks
Neural networks, particularly deep learning models, have gained traction in various fields, including employment discrimination analysis. These models consist of layers of interconnected nodes, or neurons, which can capture intricate patterns in data.
Deep learning models are capable of handling large, unstructured datasets, such as text and images, making them suitable for complex discrimination cases. Natural language processing (NLP) techniques, when combined with neural networks, can analyze textual data, such as employee complaints or HR records, to identify potential instances of discrimination.
The challenge with deep learning models lies in their complexity and the need for substantial amounts of labeled training data. Interpretability is also a concern, as neural networks can be seen as “black boxes,” making it difficult to explain their decision-making processes. Efforts to improve interpretability and fairness in deep learning are ongoing in the field of legal AI.
Natural Language Processing (NLP)
Natural Language Processing (NLP) is a subfield of machine learning that focuses on the interaction between computers and human language. In the context of employment discrimination analysis, NLP techniques are crucial for processing and understanding textual data, such as employee complaints, emails, or legal documents.
NLP algorithms can perform tasks such as sentiment analysis to gauge the emotional tone of text, entity recognition to identify names and organizations, and topic modeling to uncover hidden themes within large text corpora. These capabilities enable legal professionals to extract valuable insights from text data, potentially revealing discriminatory practices or biased language.
However, NLP comes with its own set of challenges, including the need for labeled training data, handling unstructured text, and addressing potential biases in language models. Legal AI developers work on adapting NLP techniques to the unique requirements of employment discrimination analysis while ensuring fairness and accuracy.
Moving Forward in Legal AI and Employment Discrimination Analysis
The field of machine learning for employment discrimination analysis is continuously evolving, driven by advancements in technology and a growing awareness of the importance of diversity, equity, and inclusion in the workplace. As legal AI continues to mature, it’s crucial for legal professionals to stay informed about the latest developments and best practices in this domain.
To harness the full potential of machine learning in employment discrimination analysis, consider the following steps:
- Collaboration: Foster collaboration between legal professionals and data scientists or machine learning experts. Effective communication and a shared understanding of the legal and technical aspects are essential.
- Data Governance: Implement robust data governance practices to ensure data quality, privacy compliance, and bias mitigation. Curate diverse and representative datasets to train fair and accurate machine learning models.
- Interpretability: Explore methods for making machine learning models more interpretable. Explainable AI techniques can help legal professionals understand and trust the decisions made by algorithms.
- Ethical Considerations: Engage in discussions about the ethical implications of using machine learning in employment discrimination analysis. Consider the potential impact on individuals and society and work to strike the right balance between automation and human judgment.
- Continuous Learning: Stay updated with the latest legal AI developments and industry trends. Attend conferences, workshops, and webinars to gain insights into emerging technologies and best practices.
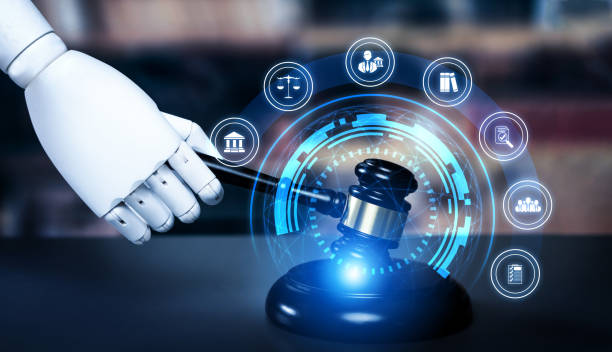
Conclusion
Machine learning for employment discrimination analysis is a promising application of legal AI that offers enhanced efficiency, improved accuracy, and data-driven insights in the legal field. However, it comes with its own set of challenges, including data bias, interpretability issues, and ethical considerations. Navigating the legal implications of using machine learning in discrimination analysis is crucial to ensuring fair and just outcomes.
As technology continues to advance, legal professionals and organizations must strike a balance between harnessing the power of machine learning and upholding the principles of justice, fairness, and accountability in employment discrimination cases. By embracing technology while remaining vigilant about its ethical and legal implications, the legal community can leverage the benefits of machine learning to promote a more equitable workplace and society as a whole.
In conclusion, machine learning holds immense promise in the realm of employment discrimination analysis, offering legal professionals valuable tools to enhance efficiency, accuracy, and fairness in addressing discrimination cases. By navigating the challenges and ethical considerations thoughtfully, legal AI can contribute to a more equitable and just workplace for all.