Generative AI has had a profound effect on various areas of our lives. From voice assistants like Siri and Alexa to customer service chatbots on websites, AI is now part of everyday online interactions.
Generative AI, including models such as GANs (Generative Adversarial Networks) and VAEs (Variational Autoencoders), is expected to have a significant impact on intellectual property (IP) in the future.
With its ability to create original content, Generative AI poses both opportunities and challenges in the realm of intellectual property (IP). Let’s explore the future impact of generative AI on IP-related opportunities and challenges.
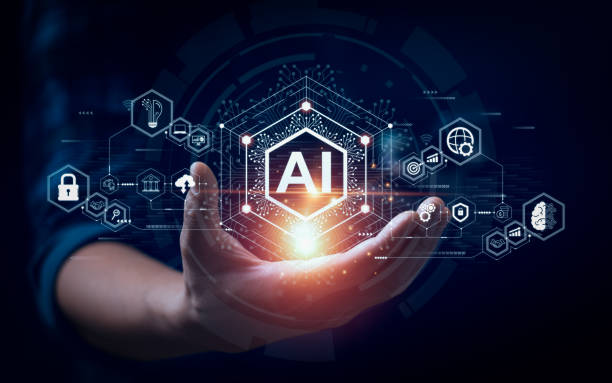
Future Impact of Generative on Intellectual Property(IP)
Generative Artificial Intelligence (AI) has emerged as a powerful tool, capable of autonomously creating original content across various domains.
While its potential for innovation and creativity is immense, the future impact of generative AI on intellectual property (IP) raises important questions. As generative AI becomes more sophisticated, it challenges existing frameworks of copyright, ownership, and the ethical considerations surrounding AI-generated works.
Opportunities that Generative AI provides
1. Improved Productivity
Generative AI allows workers to spend less time on routine, repetitive tasks and devote their efforts to more challenging and creative aspects of their jobs, potentially helping organizations produce and distribute more content faster than ever before.
Deep learning’s rapid advancement makes this possible. While traditional rules-based algorithms can perform predictable tasks such as text parsing and voice recognition, more sophisticated models using generative neural networks have seen rapid advancement.
Gradient descent-based methods like GANs and VAEs enable faster data modeling, compression, generation, and compression/generation processes which speed up generative AI’s transformation of raw data into content critical to business success more rapidly than ever.
Generative models also can handle more diverse data types, making them an invaluable asset to businesses that require specific types of content for specific use cases. For instance, creating 3D assets manually can be time-consuming and costly.
Generative models provide a way around this by automatically building and analyzing an extensive variety of designs to streamline this process.
Generative models can create text, images, music clips, and other forms of digital content using different inputs and outputs, making them an invaluable tool for creating documents, proofreading text, or designing sample designs – sometimes producing content that cannot be distinguished from human-created work.
2. Higher Productivity Across the Value Chain
Recent advances in Generative AI have been remarkable. ChatGPT, a large language model (LLM), has captured the hearts and minds of hundreds of millions around the globe and marked AI’s first true inflection point for mass adoption.
Furthermore, LLMs and foundation models – vast systems composed of deep neural networks – can now quickly be fine-tuned for multiple tasks creating immense productivity gains along the value chain.
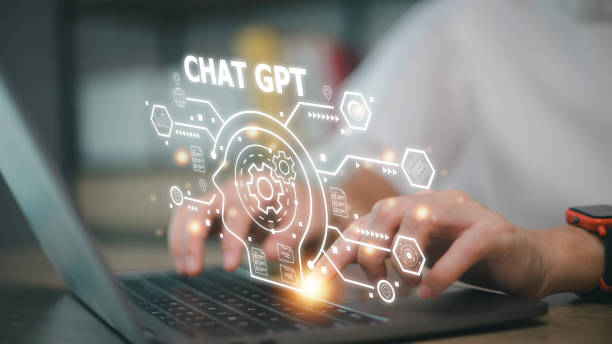
Generative AI’s impact on business process work can be immense. A recent study concluded that it improved call center agent productivity by 14%. Such automation enables agents to focus more effectively on higher-value work while improving customer service outcomes.
Other studies indicate that Generative AI could bring with it a wide range of new digital services, from virtual reality experiences and the monetization of user-generated content (UGC) to automating much of the work associated with data management, giving enterprises greater agility, cost savings, and operational efficiencies.
Generative AI can also assist businesses with limited training data to develop more robust and effective AI applications. For instance, generative AI systems could use existing contracts, proposals, and other documentation to extrapolate potential risk for new procurement processes; or generate synthetic data automatically in order to train quality systems that detect product flaws early during production cycles.
Organizations looking to maximize the benefits of Generative AI should act swiftly and with an enterprise-minded mindset, starting by identifying consumable models and applications that can be implemented quickly with instantaneous results, before investing in job redesign, skill upskilling, reinvention using tailored models customized specifically to their data.
3. Improved Customer Experience
Generative AI’s ability to analyze data and produce high-quality responses makes it ideal for use in customer service applications.
A contact center could use this type of AI to provide agents with tailored “best next action” recommendations for each customer based on their context and historical data – this could improve customer experience by eliminating unnecessary wait times while offering more tailored, targeted responses.
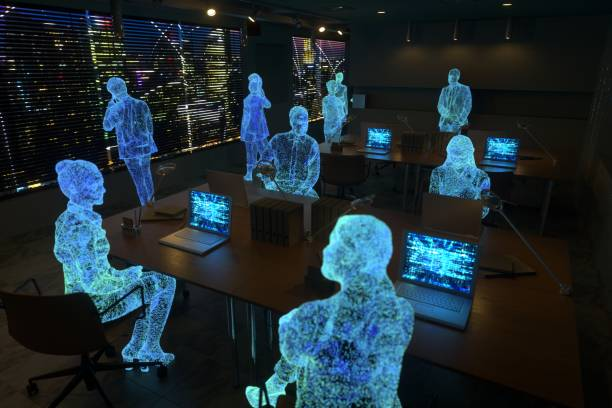
4. Increased Innovation
Generative AI empowers all individuals to express their creativity. This allows for faster experimentation of new ideas, opening up entirely new business opportunities. As this technology becomes increasingly widespread, it is vital that its benefits are distributed equally and that workers possess the skills needed to maximize its potential.
Generative AI tools differ from traditional machine learning models in that they ingest content from the Internet and use it to predict or generate outputs, potentially leaving it open to errors and biases. A text model such as GPT-3 requires massive amounts of raw input data from various parts of the Internet; something an ordinary startup cannot afford.
Businesses looking to use generative AI successfully should take two approaches when experimenting with it: first, using low-hanging fruit applications and foundation models that produce quick returns; and second, adapting their research, creative or engineering workflows using AI by replacing manual steps with algorithms and automating data processing and labeling tasks.
However, to minimize risks related to using these tools, businesses must also ensure they have an adequate talent and innovation strategy – this way they can fully take advantage of generative AI’s capabilities while pushing the envelope on what their industries can achieve.
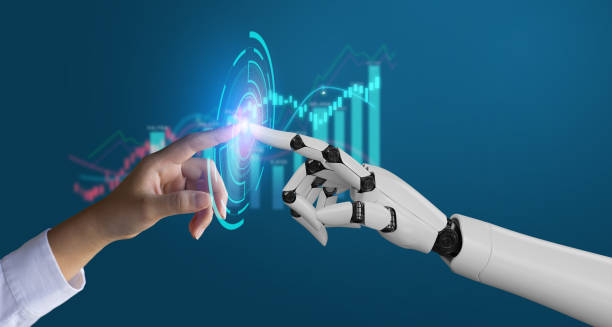
5. Higher Productivity in R&D
Generative AI is revolutionizing R&D teams’ work. Thanks to its ability to generate new ideas, it is making it easier for R&D teams to identify and pursue opportunities more easily, saving both time and resources. Furthermore, its fast prototyping process speeds up. For instance, Brynjolfsson, Li, & Raymond (2023) conducted research that revealed call center operators who used generative AI as an aid were 14% more productive due to this technology capturing tacit knowledge on solving customer problems that is usually shared through on-the-job training sessions.
However, productivity gains don’t come without risk. Like other technological innovations that have changed jobs in the past, Generative AI may replace some tasks and force workers into new roles with lower wages – Eloundou et al’s paper predicts that as many as 49% of current workers may lose half or more of their job tasks in response. This would especially reverberate among lower-skilled employees whose skills are less valued in the marketplace.
Leaders must ensure employees understand how best to utilize generative AI.
Furthermore, they should implement processes to monitor how this technology is being utilized and retrain staff as necessary – this will reduce the chances of employees using it outside their expertise and using public chatbots for sensitive information sharing – this creates security risks while jeopardizing data integrity within an organization.
6. Licensing and Monetization Models
As generative AI gains prominence, new licensing and monetization models may emerge. Creators of generative AI models could license their models or sell access to them, enabling others to generate content based on their capabilities. This creates opportunities for revenue generation, but it also requires defining appropriate licensing frameworks that consider the rights of both AI developers and content creators.
7. Redefining Creativity and Collaboration
Generative AI has the potential to transform the creative process and foster new forms of collaboration. Artists, designers, and content creators can collaborate with generative AI systems, pushing the boundaries of traditional IP and exploring new creative territories.
This requires reevaluating the concept of authorship, understanding the rights and responsibilities of human-AI collaborations, and developing new licensing and ownership frameworks.
Challenges
1. Copyright Ownership and Attribution
Generative AI blurs the lines of authorship and ownership, making it difficult to determine the rightful owner of AI-generated works. Clear guidelines and regulations are needed to address attribution challenges and establish copyright ownership for content created by AI systems.
2. Plagiarism and Infringement
Generative AI models trained on large datasets, including copyrighted works, may unintentionally produce content that resembles existing creations. This raises concerns about plagiarism and infringement. Developing effective methods to distinguish between original and AI-generated content is crucial for protecting the rights of content creators.
3. Identifying Originality
With the advancement of generative AI, distinguishing between original works and AI-generated replicas becomes increasingly challenging. This poses difficulties in determining the novelty and creativity required for copyright protection, leading to potential disputes over the originality of AI-generated content.
4. Licensing and Monetization
As generative AI becomes more prevalent, new licensing and monetization models will need to be developed. Determining fair compensation for AI-generated content, establishing licensing agreements, and ensuring appropriate revenue distribution among AI developers, content creators, and other stakeholders will require innovative approaches and legal frameworks.
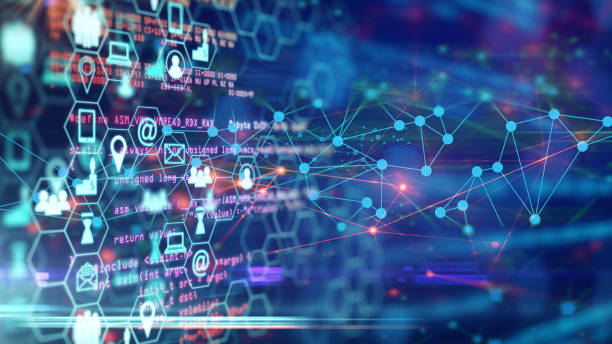
5. Bias and Discrimination
Generative AI models learn from training data, which can introduce biases and discriminatory patterns into the generated content. Addressing bias in AI algorithms and ensuring fairness in AI-generated works are important considerations to prevent perpetuating societal inequalities and discrimination.
6. Privacy and Data Protection
Generative AI models often require large datasets, potentially including personal or sensitive information. Safeguarding user privacy, complying with data protection regulations, and ensuring secure handling of training data are essential to prevent misuse and unauthorized access to personal information.
7. Regulatory Frameworks and Standards
The rapid development of generative AI necessitates the establishment of robust regulatory frameworks and standards. These frameworks should address IP issues related to generative AI, providing guidelines for copyright protection, licensing, attribution, and fair use in the context of AI-generated content.
8. Ethical Guidelines and Accountability
Generative AI raises ethical concerns, including the potential for manipulation, deception, and the misuse of AI-generated content. Establishing ethical guidelines for AI development and usage, ensuring transparency, and holding AI developers accountable for the outcomes of their AI systems are vital for building trust and mitigating potential risks.
Conclusion
Generative AI holds tremendous potential to revolutionize various industries and drive innovation. However, its future impact on intellectual property raises complex challenges that must be addressed to strike a balance between encouraging creativity, protecting original works, and adapting to the evolving technological landscape.
Navigating these challenges requires a collaborative approach involving stakeholders from various domains, including technology, law, ethics, and policy.
By addressing these challenges, we can unlock the full potential of generative AI while ensuring the protection of intellectual property rights and fostering innovation in a rapidly evolving landscape.