In the fast-paced world of technology and innovation, securing intellectual property rights has become paramount. Patents, in particular, play a pivotal role in safeguarding inventive concepts and ideas. A patent landscape is an analysis of patent data that reveals business, scientific, and technological trends.
Landscape reports typically focus on a single geographic region, industry, or technology However, navigating the intricate web of patents, identifying emerging trends, and understanding the competitive landscape has always been a challenging task for innovators, researchers, and businesses. That’s where Artificial Intelligence (AI) comes into play.
Understanding Patent Landscapes
Before we dive into the transformative power of AI in patent analysis, let’s establish a foundational understanding of patent landscapes. A patent landscape is essentially a visual representation of the patent landscape for a specific technology or industry. It provides a comprehensive overview of the patents related to a particular domain, showcasing key players, technology trends, and potential opportunities and threats. In essence, it’s a roadmap for innovators and businesses to navigate the complex world of patents.
The significance of patent landscapes cannot be overstated. They serve as strategic decision-making tools, offering insights that can shape the future of innovation. Here’s why patent landscapes matter:
The Evolution of Patent Analysis
Traditionally, patent analysis involved hours of manual research, poring over patent documents, and sifting through vast amounts of data. However, this approach had limitations in terms of efficiency, accuracy, and scalability.
Traditional Patent Analysis Methods
In the pre-AI era, patent analysis primarily relied on human expertise and manual processes. Analysts would manually review patent documents, categorize them, and extract relevant information. While this approach yielded valuable insights, it was time-consuming, error-prone, and limited in its ability to handle large datasets.
Limitations of Manual Patent Analysis
Manual patent analysis had several drawbacks, including:
Introduction of AI in Patent Analysis
The advent of Artificial Intelligence (AI) marked a revolutionary turning point in patent analysis. AI-powered tools and techniques brought unprecedented efficiency, accuracy, and scalability to the process.
Machine Learning Algorithms
Machine learning algorithms have played a pivotal role in AI-driven patent analysis. These algorithms can categorize patents, identify trends, and make predictions based on vast datasets. For example, classification algorithms can group patents by technology domain, while predictive analytics can forecast future trends.
Natural Language Processing (NLP)
Natural Language Processing (NLP) is another critical component of AI in patent analysis. NLP enables computers to understand and extract insights from text data, such as patent documents. It can perform tasks like text mining and sentiment analysis, helping analysts gain a deeper understanding of the content.
Big Data and Cloud Computing
The marriage of AI with big data and cloud computing further amplified the capabilities of patent analysis. Researchers and businesses can now analyze massive patent datasets quickly and efficiently, thanks to cloud-based AI solutions.
Benefits of AI-Driven Patent Landscape Analysis
The integration of AI into patent analysis has brought about a multitude of benefits, making it an indispensable tool for businesses and innovators.
Efficiency and Speed
AI-driven patent analysis can process vast amounts of patent data in a fraction of the time it would take for manual analysis. This accelerated pace allows businesses to stay ahead of rapidly evolving industries. AI algorithms are not susceptible to human errors or biases. They can consistently and accurately classify patents, extract relevant information, and identify patterns that might elude human analysts. With AI, scalability ceases to be a concern. Whether you’re dealing with thousands or millions of patents, AI-powered systems can handle the workload efficiently.
Discovering Hidden Insights
AI’s ability to analyze unstructured data means that it can uncover hidden insights buried within patent documents. These insights can reveal emerging technologies and competitive strategies that might otherwise remain hidden. AI-driven systems can provide real-time updates on patent activity within a specific technology domain. This real-time monitoring enables businesses to adapt quickly to changes in the competitive landscape.
Key AI Technologies in Patent Analysis
To appreciate the full scope of AI’s impact on patent analysis, it’s essential to understand the key AI technologies that drive this transformation.
Machine Learning Algorithms
Classification and Clustering: Machine learning algorithms can automatically classify patents into categories based on their content. Clustering algorithms group similar patents together, helping analysts identify technology trends.
Predictive Analytics: Machine learning models can forecast future trends in patent activity, enabling businesses to make proactive decisions.
Natural Language Processing (NLP)
Text Mining: NLP techniques can extract valuable information from patent documents, including technical details, keywords, and inventors’ names.
Sentiment Analysis: NLP can assess the sentiment expressed in patents, helping businesses gauge the competitive landscape and potential opportunities.
Computer Vision
Image Recognition: In addition to text data, AI can analyze patent figures and drawings using computer vision, providing a holistic view of patent content.
Visualization: AI-powered visualization tools can create interactive charts and graphs that make complex patent data more accessible and understandable.
Data Integration and Knowledge Graphs
AI can integrate patent data with other sources of information, such as scientific publications and market reports, to provide a richer context for patent analysis. Knowledge graphs help map the relationships between patents, technologies, and inventors.
Steps in AI-Driven Patent Landscape Analysis
Now that we understand the benefits and key technologies, let’s delve into the practical steps involved in AI-driven Patent Landscape Analysis.
1. Data Acquisition
The journey begins with data acquisition. Analysts need access to patent databases, which are vast repositories of patent documents. Additionally, non-patent literature, such as scientific papers and industry reports, can provide valuable context.
2. Pre-Processing and Data Cleaning
Raw patent data often requires pre-processing and cleaning. This step involves removing noise, standardizing formats, and ensuring data quality.
3. AI-Powered Analysis
This is where the true magic of AI happens:
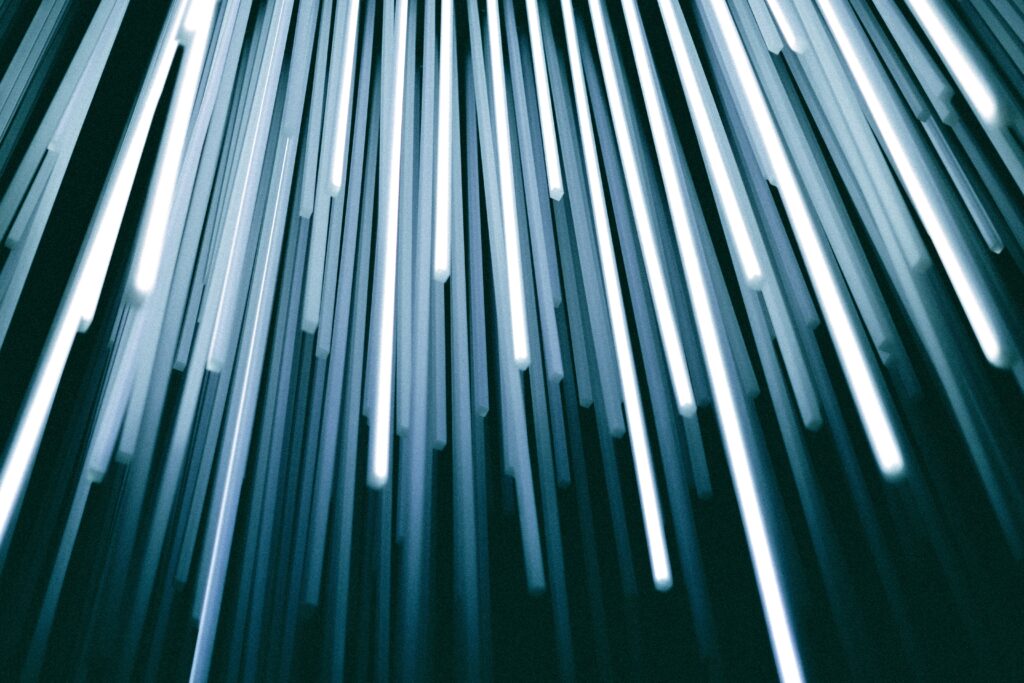
4. Visualization and Reporting
The insights derived from AI-driven analysis need to be presented in a digestible format:
5. Interpretation and Decision-Making
The final step involves interpreting the AI-generated insights and using them to inform strategic decisions. This may include adjustments to R&D strategies, IP protection plans, or competitive positioning.
Use Cases and Applications
AI-driven Patent Landscape Analysis has found applications across various industries and is transforming the way businesses approach innovation and intellectual property.
Industry-Specific Examples
Pharmaceutical: In the pharmaceutical industry, AI-driven analysis can identify emerging drug technologies, potential collaborations, and competitive threats. This information is critical for drug development and patent strategy.
Technology: In the technology sector, tracking patent activity is essential for staying competitive. AI can help identify areas where innovation is thriving and areas where it’s lacking.
Automotive: AI analysis of the automotive patent landscape can uncover trends in electric vehicle technology, autonomous driving, and sustainable materials, guiding companies in their R&D efforts.
Strategic Applications
Product Development: By understanding the patent landscape, businesses can develop products that are less likely to infringe on existing patents, reducing legal risks.
IP Strategy: AI-driven analysis helps businesses formulate robust IP strategies, including decisions on patent filing, licensing, and litigation.
Licensing and Partnerships: Companies can use patent landscape insights to identify potential licensing opportunities or partnership prospects, accelerating innovation.
Litigation Support: In patent litigation cases, AI can assist legal teams by providing a comprehensive analysis of relevant patents and prior art.
Challenges and Ethical Considerations
While AI-driven Patent Landscape Analysis offers immense benefits, it’s not without its challenges and ethical considerations. AI analysis requires access to patent data, which raises concerns about data privacy and security. Protecting sensitive information in patent documents is paramount.
AI algorithms are only as good as the data they’re trained on. If the training data contains biases, AI can perpetuate those biases in its analysis. This can lead to unfair advantages or disadvantages for certain inventors or technologies.
Intellectual Property Concerns
As AI-generated innovations become more common, questions arise about the ownership of patents generated by AI systems. Legal frameworks need to adapt to address these emerging challenges. Balancing the roles of human experts and AI systems in patent analysis is an ongoing challenge. While AI can automate many tasks, human expertise remains invaluable in interpreting results and making strategic decisions.
Future Trends and Developments
The future of AI-driven Patent Landscape Analysis is promising, with several trends and developments on the horizon. AI is continually evolving, with improvements in machine learning algorithms, NLP, and computer vision. These advancements will enhance the capabilities of patent analysis tools.
AI-driven patent analysis is likely to integrate with other emerging technologies, such as blockchain, to enhance security and transparency in patent management. As AI plays a more significant role in intellectual property management, regulatory frameworks will need to adapt to ensure fairness, transparency, and accountability.
The widespread adoption of AI in patent analysis will have a profound impact on innovation and the intellectual property landscape. It will influence how businesses approach R&D, patent strategy, and competition.
AI-driven Patent Landscape Analysis represents a seismic shift in how businesses and innovators approach patent analysis. It offers unparalleled efficiency, accuracy, and scalability, empowering decision-makers to navigate the complex world of patents with confidence.
As we move forward, it’s essential for businesses and researchers to embrace AI as an indispensable tool in their intellectual property strategies. By harnessing the power of AI, they can stay at the forefront of innovation, secure their intellectual property rights, and drive advancements in technology and industry.
The transformative potential of AI in shaping the future of innovation and intellectual property is undeniable. It’s a journey worth embarking on—one that promises to redefine the way we innovate and protect our creative endeavors.