Introduction
Artificial Intelligence (AI) has become a revolutionary force across numerous industries, and the field of law is no exception. Gone are the days when lawyers pored over endless documents and precedents to assess the potential outcomes of legal cases. Today, AI is ushering in a new era of legal practice, where data-driven insights are helping legal professionals make more informed decisions. In this article, we will delve into the fascinating realm of AI-driven Legal Case Outcome Analysis, exploring the intricacies, benefits, challenges, and future prospects of this transformative technology.
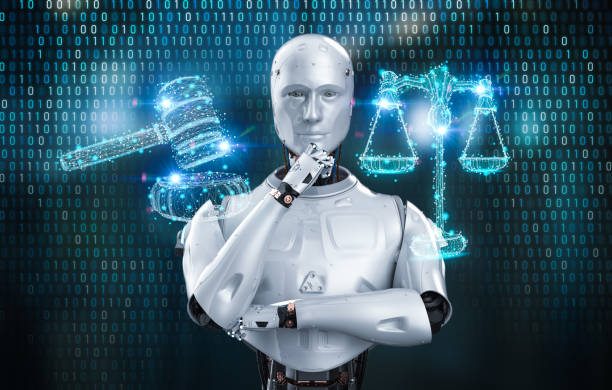
The Role of AI in Legal Case Analysis
The legal profession is often associated with vast amounts of data, including statutes, regulations, legal precedents, and case documents. Analyzing this data manually can be a time-consuming and error-prone process. This is where AI steps in, offering the ability to process and interpret vast datasets with speed and precision.
AI’s Transformational Impact on Legal Case Analysis
AI is revolutionizing legal case analysis in several ways:
- Efficiency: AI-powered tools can review and extract relevant information from thousands of legal documents in a fraction of the time it would take a human. This enables lawyers to focus on higher-level tasks.
- Predictive Analysis: AI algorithms can analyze historical case data to predict likely case outcomes. By identifying patterns and trends, AI can assist lawyers in developing winning strategies.
- Legal Research: Legal research tools powered by AI can provide lawyers with instant access to a vast repository of legal knowledge, helping them find relevant cases, statutes, and regulations.
- Document Review: AI can be used to review documents for relevance and privilege, streamlining the discovery process in litigation.
- Risk Assessment: AI can assess the risk associated with specific legal strategies, enabling lawyers to make informed decisions about how to proceed.
The benefits of AI in legal case analysis are undeniable, but to understand its workings better, let’s explore the core AI technologies driving these advancements.
AI Technologies for Legal Case Analysis
AI’s prowess in legal case analysis relies on several key technologies:
1. Natural Language Processing (NLP)
NLP in Legal Contexts
Natural Language Processing (NLP) is a branch of AI that focuses on the interaction between computers and human language. In legal contexts, NLP plays a crucial role in understanding and extracting valuable insights from legal documents.
Consider the immense volume of text in legal cases, from contracts to court decisions. NLP algorithms can analyze this text, identify relevant clauses or arguments, and categorize information.
Applications of NLP in Legal Case Analysis
- Legal Document Summarization: NLP can automatically summarize lengthy legal documents, making it easier for lawyers to grasp the key points.
- Contract Analysis: NLP tools can review contracts, highlighting critical terms and potential risks.
- Sentiment Analysis: NLP can analyze public sentiment and media coverage, providing insights into how a case is perceived by the public.
- Legal Chatbots: NLP-driven chatbots can answer common legal queries, offering valuable assistance to clients and saving lawyers time.
2. Predictive Analytics
How Predictive Analytics Works in Legal Cases
Predictive analytics involves using historical data to predict future outcomes. In legal case analysis, this means analyzing past cases to determine the likelihood of success in a current case.
These algorithms consider various factors, including case type, jurisdiction, judge, and even external factors such as economic conditions or political climate.
Examples of Predictive Analytics Tools
Several software platforms specialize in predictive analytics for legal cases:
- Lex Machina: This platform uses data analysis to predict litigation outcomes, offering insights into judge behavior and case trends.
- Ravel Law: Ravel Law’s software provides data-driven insights into case law, helping lawyers understand how judges rule on specific issues.
- ROSS: ROSS uses AI to assist lawyers in legal research, offering predictive suggestions for relevant cases and statutes.
3. Machine Learning Algorithms
Overview of Machine Learning in Legal Case Analysis
Machine learning algorithms play a vital role in AI-driven legal case analysis. These algorithms can be trained on historical legal data to recognize patterns, make predictions, and provide valuable recommendations.
Consider a scenario where a law firm wants to predict the outcome of a personal injury case. Machine learning algorithms can analyze past personal injury cases, taking into account factors such as the type of injury, location, and legal precedents.
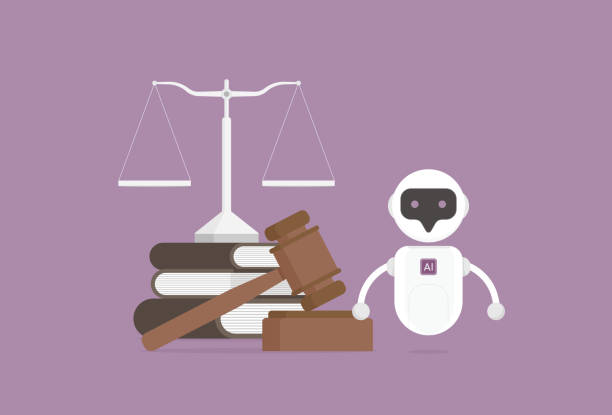
Case Studies Showcasing the Use of Machine Learning
To illustrate the practical application of machine learning in legal case analysis, let’s explore a couple of case studies:
Case Study 1: Predicting Patent Infringement Outcomes
In intellectual property law, patent infringement cases can be complex and costly. A law firm specializing in patent litigation used machine learning to predict the outcomes of such cases.
By feeding historical case data into their machine learning model, the firm was able to identify factors that significantly influenced case outcomes. These factors included the strength of the patent, the defendant’s history of infringement, and the judge’s track record in patent cases.
The model’s predictions were remarkably accurate, allowing the firm to advise clients more effectively on whether to pursue or settle cases.
Case Study 2: Streamlining Immigration Case Processing
Immigration law firms often face high volumes of cases, each with unique circumstances. One firm employed machine learning to streamline their case processing.
The machine learning model categorized incoming immigration cases based on factors such as visa type, country of origin, and immigration status. It then assigned priority levels to each case, helping lawyers focus on cases requiring immediate attention.
This automation reduced case processing time by 30%, enabling the firm to provide faster and more efficient service to clients.
Data Collection and Preprocessing
Now that we’ve explored the core AI technologies used in legal case analysis and seen their practical applications, it’s crucial to understand the importance of data in AI-driven analysis and how it’s prepared for use.
Importance of Quality Data in AI-driven Legal Analysis
Quality data is the lifeblood of AI-driven legal analysis. Without accurate and relevant data, AI algorithms would be ineffective. In legal case analysis, data encompasses various sources, including case documents, court records, and legal databases.
The success of AI models in predicting legal outcomes depends on having access to comprehensive and up-to-date data. Data quality issues can lead to erroneous predictions and undermine the value of AI in the legal field.
Data Sources for Legal Case Analysis
- Legal Databases: Legal research databases such as Westlaw and LexisNexis provide a vast repository of legal documents and case law.
- Court Records: Access to court records is essential for obtaining information about case history, judgments, and rulings.
- Client Data: Law firms can leverage their own case data to train AI models, provided they have the necessary permissions and safeguards in place.
- Public Records: Some legal information, such as property records or business registrations, can be obtained from publicly available sources.
Data Preprocessing Techniques
Once data is collected, it must undergo preprocessing to make it suitable for AI analysis. Data preprocessing involves several steps:
1. Data Cleaning
Data cleaning involves removing or correcting errors, inconsistencies, and outliers in the dataset. This step is crucial to ensure the accuracy of AI models. Common data cleaning tasks include:
- Removing duplicate records
- Handling missing data through imputation or deletion
- Correcting data format errors
- Addressing data entry errors
2. Data Integration
Legal case analysis often involves combining data from multiple sources. Data integration ensures that different datasets are compatible and can be used together. This step may require mapping data to a common schema and resolving discrepancies in data structure.
3. Data Transformation
Data transformation involves converting data into a suitable format for analysis. This may include:
- Scaling numerical features
- Encoding categorical variables
- Creating new features through feature engineering
By the end of the data preprocessing phase, the dataset should be clean, well-structured, and ready for use in AI model development.
Building AI Models for Legal Case Outcome Analysis
With high-quality data in hand, the next step in AI-driven legal case analysis is building and training AI models. The process involves several key considerations and steps:
1. The Role of Feature Selection and Engineering
In AI, features are the variables or attributes used to make predictions. Feature selection involves choosing the most relevant features for a particular analysis. Legal case analysis often involves selecting features that have a significant impact on case outcomes.
Feature engineering, on the other hand, involves creating new features or transforming existing ones to improve the performance of AI models. For example, in a personal injury case analysis, feature engineering might involve calculating the severity of an injury based on medical reports or estimating the defendant’s financial resources.
Both feature selection and engineering are essential to ensure that AI models are capable of making accurate predictions.
2. Model Training and Validation
Model training is the process of teaching an AI algorithm to recognize patterns and make predictions based on historical data. During training, the algorithm adjusts its parameters to minimize prediction errors.
Validation is a crucial step to assess the model’s performance. It involves testing the trained model on a separate dataset that it has never seen before. This helps ensure that the model generalizes well to new cases.
Legal case analysis often requires the use of supervised learning, where the model is trained on labeled data—data with known outcomes. For instance, the model may be trained to predict whether a contract is likely to be upheld in court based on historical contract cases with known verdicts.
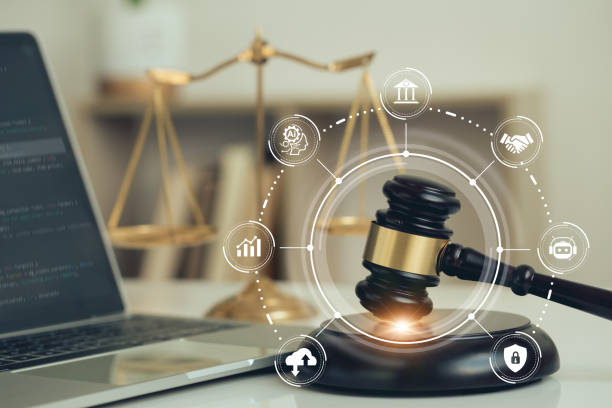
Ethical Considerations in AI Model Development
As AI becomes more integrated into legal practice, ethical considerations take on increasing importance. Lawyers and AI developers must address ethical concerns related to AI-driven legal case analysis, including:
- Bias and Fairness: AI models can inadvertently perpetuate biases present in historical data. It’s crucial to identify and mitigate bias to ensure that AI-driven analyses are fair and equitable.
- Privacy and Confidentiality: AI analysis often involves sensitive legal documents. Ensuring the privacy and confidentiality of client information is paramount.
- Transparency: AI models should be transparent in their decision-making processes. Lawyers and clients need to understand how AI arrives at its predictions.
- Accountability: Who is responsible for AI-driven decisions in legal cases? Establishing accountability is essential, especially when AI recommendations influence case strategy.
Future Trends in AI-Driven Legal Case Analysis
As AI continues to evolve, several trends are shaping the future of AI-driven legal case analysis:
1. Increased Adoption of AI Chatbots
AI chatbots are becoming commonplace in law firms and legal departments. They assist with client inquiries, document drafting, and other routine tasks, freeing up lawyers to focus on more complex matters.
2. Enhanced Predictive Analytics
Advancements in machine learning and data analysis will lead to even more accurate predictive analytics models. Lawyers will rely on these models to assess case outcomes and develop winning strategies.
3. Improved Natural Language Processing
NLP technology will become more sophisticated, enabling AI systems to understand and interpret legal language with greater precision. This will facilitate more accurate legal research and document analysis.
4. Ethical AI Development
The legal profession will continue to prioritize ethical AI development, with a focus on addressing bias, ensuring transparency, and upholding data privacy and security standards.
5. Regulatory Frameworks
Regulatory bodies will establish guidelines and standards for the responsible use of AI in the legal industry. These frameworks will provide legal professionals with clear guidance on AI adoption and usage.
Conclusion
AI-driven Legal Case Outcome Analysis is reshaping the legal landscape, offering unprecedented efficiency, data-driven insights, and cost savings. Real-world case studies demonstrate the tangible benefits of AI across criminal and civil law cases. However, ethical considerations and transparency remain critical as AI’s role in the legal profession expands. As we navigate the ever-evolving intersection of AI and law, it’s essential for legal professionals to embrace AI as a powerful tool while upholding the core principles of fairness, privacy, and justice.