Introduction
In an era defined by technological advancements and innovation, the protection of intellectual property rights has never been more critical. Patents, in particular, play a pivotal role in safeguarding innovations and ensuring that creators and inventors are duly rewarded for their contributions. However, the sheer volume of patent data generated worldwide is staggering, making it increasingly challenging for individuals, businesses, and patent offices to efficiently manage, analyze, and make informed decisions regarding patents. This is where the marriage of patent data analytics and machine learning comes into play.
In this comprehensive exploration, we will delve deep into the realm of patent data analytics, exploring how machine learning techniques are transforming the way we understand, utilize, and protect intellectual property. We will unravel the intricacies of patent data, discuss the role of machine learning algorithms, and highlight real-world applications of this dynamic synergy. By the end of this journey, you’ll have a profound understanding of how patent data analytics with machine learning is reshaping the landscape of intellectual property law.
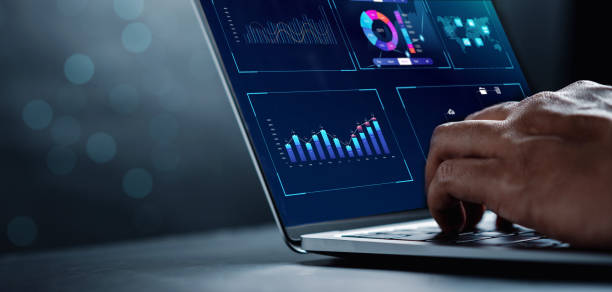
The Immense World of Patent Data
Patent Data Overload
The World Intellectual Property Organization (WIPO) reported that in 2019 alone, over 3.3 million patent applications were filed worldwide. This vast reservoir of patent data includes descriptions, claims, citations, and much more. It’s a treasure trove of innovation, but also an overwhelming challenge for patent offices, researchers, and businesses alike.
The sheer volume of patent data can be likened to a labyrinthine library without a catalog, making it difficult to extract meaningful insights or navigate effectively. This is where the integration of machine learning into patent data analytics proves to be revolutionary.
The Role of Patent Offices
Patent offices, like the United States Patent and Trademark Office (USPTO), are tasked with examining and granting patents. However, their responsibilities extend beyond this core function. Patent offices are custodians of patent data, responsible for ensuring that it is accurate, accessible, and can withstand legal scrutiny. The USPTO, in particular, serves as a vital reference point for patent data in the United States.
The USPTO’s role in patent data management involves not only granting patents but also maintaining a comprehensive database of granted patents, published applications, and historical records. This database is a goldmine for researchers, inventors, and innovators, serving as a primary source of information for patent data analytics.
Unleashing Machine Learning on Patent Data
Understanding Machine Learning
Before we dive into how machine learning is transforming patent data analytics, let’s establish a foundational understanding of what machine learning entails.
Machine Learning Basics
Machine learning is a subfield of artificial intelligence (AI) that focuses on the development of algorithms and statistical models. These algorithms enable computers to learn and make predictions or decisions without being explicitly programmed. In essence, machine learning allows computers to recognize patterns, draw insights from data, and improve their performance over time.
Supervised vs. Unsupervised Learning
In the context of patent data analytics, two primary categories of machine learning are particularly relevant: supervised and unsupervised learning.
Supervised Learning: In supervised learning, the algorithm is trained on labeled data, where the outcome or target variable is known. This type of learning is often used for tasks such as classification, where the algorithm learns to categorize data into predefined classes or labels. For instance, it can be used to classify patents into different technology categories or determine patent validity.
Unsupervised Learning: Unsupervised learning, on the other hand, deals with unlabeled data. The algorithm identifies patterns or structures in the data without specific guidance. Clustering, a common unsupervised learning technique, can be applied to group similar patents together based on their content or characteristics.
The Power of Natural Language Processing (NLP)
Within the realm of machine learning, Natural Language Processing (NLP) holds a special place when it comes to patent data analytics. NLP focuses on the interaction between computers and human language, enabling machines to understand, interpret, and generate human-like text.
Patent Text Analysis with NLP
Patent documents are rich in text, containing detailed descriptions, claims, and references. NLP algorithms can extract valuable information from this textual data, allowing for advanced analysis.
Text Classification: NLP can be used to classify patents into various categories or identify their technological focus. For example, it can determine whether a patent pertains to biotechnology, electronics, or pharmaceuticals by analyzing the language used in the document.
Patent Prior Art Search: NLP algorithms can streamline the process of searching for prior art, which is crucial to assessing the novelty of a patent application. By analyzing the text of existing patents, NLP can help patent examiners and inventors identify relevant prior art more efficiently.
Semantic Analysis: NLP can also perform semantic analysis to understand the meaning and context of patent text. This is particularly useful in identifying potential infringements or assessing the scope of patent claims.
Predictive Analytics with Machine Learning
One of the most valuable applications of machine learning in patent data analytics is predictive analytics. Predictive models can provide insights into future trends, patent value, and potential legal issues.
Predicting Patent Grants
Machine learning models can be trained on historical patent data to predict the likelihood of a patent being granted. These models consider various factors, including the type of invention, the prior art landscape, and the quality of the patent application. Such predictions can help inventors and businesses make informed decisions about pursuing patent protection.
Assessing Patent Valuation
Determining the value of a patent is a complex task, but machine learning can simplify it. By analyzing factors such as the number of citations a patent receives, the industries it impacts, and its legal status, machine learning models can estimate the potential value of a patent. This information is invaluable for licensing negotiations, mergers, and acquisitions.
Identifying Legal Risks
Machine learning can analyze patent portfolios to identify potential legal risks and vulnerabilities. It can flag patents that may be susceptible to infringement challenges or those that overlap with existing patents. This proactive approach allows patent owners to address issues before they escalate into costly legal battles.
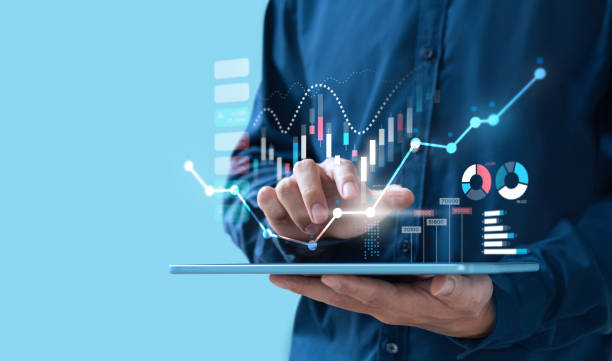
Real-world applications of Patent Data Analytics with Machine Learning
1. Intellectual Property Management
Automated Portfolio Analysis
Intellectual property (IP) management involves overseeing a company’s patent portfolio, ensuring it aligns with business goals, and optimizing its value. Machine learning can automate portfolio analysis by continuously monitoring patent data and evaluating its relevance to the company’s strategic objectives.
For instance, a pharmaceutical company can use machine learning to track emerging technologies and competitor patents. When a relevant patent is identified, the system can trigger actions such as initiating licensing negotiations or adjusting research and development priorities.
Risk Mitigation
In the realm of IP management, risk mitigation is paramount. Machine learning can help identify and mitigate risks associated with patent infringement or challenges to existing patents.
By continuously monitoring patent landscapes and legal precedents, machine learning models can provide early warnings of potential legal threats. This enables proactive measures, such as strengthening patents, licensing negotiations, or even litigation preparedness.
2. Prior Art Search and Patent Examination
Accelerated Prior Art Search
Patent examiners at organizations like the USPTO face the daunting task of conducting prior art searches to assess the novelty of patent applications. Machine learning can accelerate this process by analyzing vast amounts of textual data from existing patents and scholarly articles.
By automating the identification of relevant prior art, machine learning reduces the workload on examiners, expedites the examination process, and improves the accuracy of patent assessments.
Automated Patent Categorization
Categorizing patents into specific technological domains is essential for efficient examination and search purposes. Machine learning models can automatically categorize patents based on their content, making it easier for examiners to find relevant prior art and ensure consistent classification.
3. Patent Valuation and Licensing
Dynamic Patent Valuation
Determining the value of a patent is not a one-time task but a dynamic process influenced by various factors. Machine learning models can continuously assess a patent’s value by considering its performance metrics, market dynamics, and litigation history.
This dynamic valuation approach empowers patent owners to make data-driven decisions regarding licensing, sales, or portfolio management.
Intelligent Licensing Strategies
Licensing intellectual property can be a lucrative endeavor, but it requires strategic decision-making. Machine learning can optimize licensing strategies by analyzing historical licensing data, market trends, and competitor activities.
For instance, machine learning can recommend licensing terms, identify potential licensees, and even predict the likelihood of successful licensing negotiations, increasing the efficiency and profitability of IP monetization.
4. Litigation Support and Legal Analytics
Early Case Assessment
In the realm of intellectual property litigation, early case assessment is crucial for cost-effective and favorable outcomes. Machine learning can assist legal teams in assessing the strengths and weaknesses of their cases by analyzing vast amounts of legal documents, court decisions, and historical case data.
By providing insights into the likelihood of success, potential damages, and legal strategies employed in similar cases, machine learning enhances the decision-making process for litigation.
Predictive Legal Analytics
Machine learning can predict the outcomes of intellectual property disputes based on historical case data. These predictions can assist legal professionals in evaluating settlement options, assessing litigation risks, and optimizing legal strategies.
For example, a machine learning model can estimate the probability of winning a patent infringement lawsuit and the potential damages that could be awarded, enabling informed decisions on whether to pursue litigation or negotiate a settlement.
Challenges and Ethical Considerations
1. Data Privacy and Security
Sensitive Information
Patent data often includes sensitive information related to inventors, their inventions, and business strategies. Machine learning models must be designed with robust data privacy and security measures to protect this confidential information.
The USPTO and other patent offices have a responsibility to safeguard the data they collect and store. As they embrace machine learning for improved patent examination and analysis, ensuring the privacy and security of patent-related information is paramount.
Data Breach Risks
Machine learning models, especially those operating on cloud-based platforms, can be vulnerable to data breaches. If patent data is compromised, it can have serious consequences, including the exposure of valuable innovations or trade secrets.
It is essential for organizations, including patent offices, to implement stringent cybersecurity measures and encryption protocols to mitigate data breach risks associated with machine learning applications.
2. Bias and Fairness
Bias in Machine Learning
Machine learning models are not immune to biases, and this can be a significant concern when applied to patent data analytics. Bias can manifest in various forms, including algorithmic bias and data bias.
Algorithmic bias occurs when machine learning models reinforce or amplify existing biases present in the data they are trained on. For instance, if historical patent data exhibits gender bias, the model may perpetuate this bias when predicting patent grants or evaluating patent quality.
Data bias, on the other hand, stems from skewed or incomplete data. If certain demographics or technologies are underrepresented in the training data, the model’s predictions may be inaccurate or biased.
3. Transparency and Accountability
Explainability
Machine learning models often operate as black boxes, making it challenging to understand how they arrive at their decisions. In the context of patent data analytics, transparency is crucial for ensuring trust and accountability.
It is imperative to develop machine learning models that provide explanations for their predictions. This not only aids patent examiners, inventors, and legal professionals in understanding the basis for decisions but also helps identify and rectify any biases or errors.
Human Oversight
While machine learning can significantly enhance the efficiency and accuracy of patent data analytics, human oversight remains essential. Decisions regarding patent grants, valuation, and legal strategies must ultimately involve human judgment.
Patent offices like the USPTO must strike a balance between automation and human intervention to ensure the integrity of the patent system and uphold legal standards.
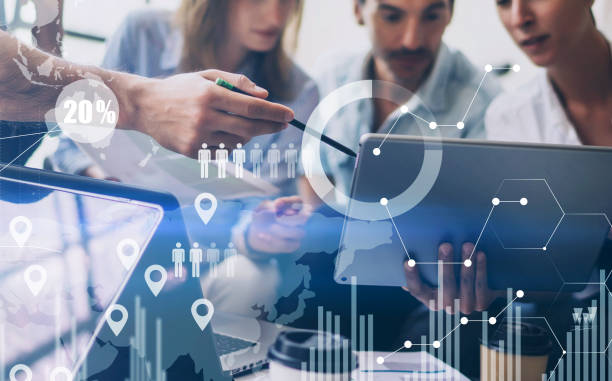
The Future of Patent Data Analytics with Machine Learning
1. Advancements in AI and ML
The field of artificial intelligence and machine learning is continually evolving, and this bodes well for the future of patent data analytics.
Advanced Algorithms
Machine learning algorithms are becoming increasingly sophisticated, enabling more precise predictions and deeper insights. Future advancements may include algorithms capable of understanding patent text in multiple languages, recognizing nuanced legal concepts, and uncovering hidden patterns in patent data.
Integration with Emerging Technologies
Machine learning will likely integrate with other emerging technologies, such as blockchain and quantum computing, to further enhance patent data analytics. For example, blockchain can provide secure and transparent patent management, while quantum computing can accelerate patent examination.
2. Enhanced Intellectual Property Protection
As machine learning becomes more integral to patent data analytics, the intellectual property protection landscape will undergo profound changes.
Faster and More Accurate Examinations
The adoption of machine learning at patent offices like the USPTO will lead to faster and more accurate patent examinations. This benefits inventors by reducing waiting times and increasing the quality of granted patents.
Improved Patent Valuation
Machine learning will continue to refine patent valuation techniques, allowing businesses to make smarter decisions regarding their patent portfolios. This will enable more efficient monetization of intellectual property.
3. Ethical and Legal Frameworks
To navigate the evolving landscape of patent data analytics with machine learning, robust ethical and legal frameworks are essential.
Regulation and Compliance
Governments and international bodies will play a pivotal role in regulating machine learning applications in intellectual property. These regulations will aim to address data privacy, bias, transparency, and accountability.
Ethical Guidelines
Professional organizations and industry groups will establish ethical guidelines for patent data analytics with machine learning. These guidelines will help practitioners uphold ethical standards and avoid unethical practices.
4. Collaboration and Knowledge Sharing
Collaboration and knowledge sharing will be at the heart of the future of patent data analytics.
International Collaboration
Given the global nature of patent data, international collaboration among patent offices and organizations will increase. This will facilitate the sharing of best practices, data, and insights.
Open Source Initiatives
Open source initiatives in the field of patent data analytics with machine learning will encourage the development of innovative tools and algorithms. These initiatives will democratize access to advanced analytics capabilities.
Conclusion
In this extensive exploration of patent data analytics with machine learning, we’ve traversed the vast landscape of patent data, examined the role of machine learning algorithms, and delved into real-world applications and challenges. The USPTO, as a key player in the world of patents, serves as a reference point for the transformational power of machine learning in intellectual property.
As we stand on the cusp of a future where patents are examined, valued, and protected with unprecedented precision and efficiency, it is imperative that we embrace the potential of machine learning while upholding ethical and legal standards. The journey of patent data analytics with machine learning is an exciting one, promising to redefine how we innovate, protect, and harness the power of intellectual property in the years to come.